Accepted posters for the workshop "UAV-based Remote Sensing Methods for Monitoring Vegetation"
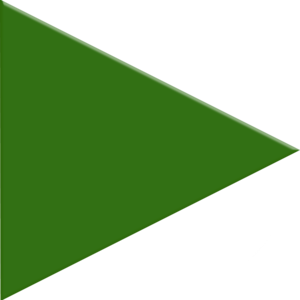
Presented by: Mr. Andreas Enders, INRES Uni Bonn
Full title: Drone flight in Agriculture - Developing a Checklist for valid scientific Drone use
Abstract: Will come soon
Keywords: Regulations for Drones, Demand for Multispectral Orthophotos
Full title: Drone flight in Agriculture - Developing a Checklist for valid scientific Drone use
Abstract: Will come soon
Keywords: Regulations for Drones, Demand for Multispectral Orthophotos
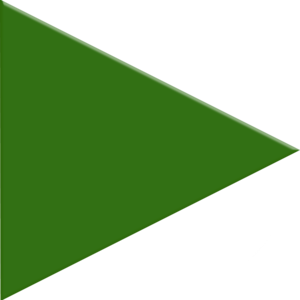
Presented by: Dr. Rene Heim, Universität Bonn
Full title: The use of multi-angular UAV remote sensing for digital plant pathology.
Abstract: Optical sensors mounted on uncrewed aerial vehicles (UAVs) are typically directed straight downward to streamline structure-from-motion and image processing. High horizontal and vertical image overlap during UAV missions leads to each object being captured from multiple view angles, thus generating a comprehensive multi-angular reflectance dataset. We have developed a method to extract reflectance data, along with their associated distinct view zenith angles (VZA) and view azimuth angles (VAA), from UAV-mounted optical cameras. This enhances plant parameter classification compared to conventional orthomosaic reflectance retrieval (https://doi.org/10.1007/s11119-024-10133-0). Currently, a follow-up project is extending this research into the field of plant pathology to explore how UAV observation angles and the seasonal dynamics of structural leaf traits influence spectral signatures and empirical modeling. At your workshop 'UAV-based Remote Sensing Methods for Monitoring Vegetation' we hope to present a poster outlining the results of the 'Applied framework for unlocking multi-angular UAV reflectance data: A case study for classification of plant parameters in maize.' We would also like to provide initial insights into the DFG project ON-Cerco, which applies the aforementioned open science framework in digital plant pathology on Cercospora beticola, responsible for the economically impactful Cercospora Leaf Spot disease in sugar beets.
Keywords: agriculture, machine learning, multispectral, open source, structural plant traits
Full title: The use of multi-angular UAV remote sensing for digital plant pathology.
Abstract: Optical sensors mounted on uncrewed aerial vehicles (UAVs) are typically directed straight downward to streamline structure-from-motion and image processing. High horizontal and vertical image overlap during UAV missions leads to each object being captured from multiple view angles, thus generating a comprehensive multi-angular reflectance dataset. We have developed a method to extract reflectance data, along with their associated distinct view zenith angles (VZA) and view azimuth angles (VAA), from UAV-mounted optical cameras. This enhances plant parameter classification compared to conventional orthomosaic reflectance retrieval (https://doi.org/10.1007/s11119-024-10133-0). Currently, a follow-up project is extending this research into the field of plant pathology to explore how UAV observation angles and the seasonal dynamics of structural leaf traits influence spectral signatures and empirical modeling. At your workshop 'UAV-based Remote Sensing Methods for Monitoring Vegetation' we hope to present a poster outlining the results of the 'Applied framework for unlocking multi-angular UAV reflectance data: A case study for classification of plant parameters in maize.' We would also like to provide initial insights into the DFG project ON-Cerco, which applies the aforementioned open science framework in digital plant pathology on Cercospora beticola, responsible for the economically impactful Cercospora Leaf Spot disease in sugar beets.
Keywords: agriculture, machine learning, multispectral, open source, structural plant traits
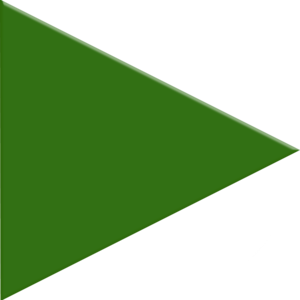
Presented by: Dr Toby Jackson, University of Bristol
Full title: How did the 2015-16 El Niño affect tropical forest restoration in Indonesia?
Abstract: Restoration of logged tropical forests is hailed as a nature-based solution to the interlinked climate and biodiversity crises, but there are concerns that recovery may be hampered by climate change. Here we use repeated airborne surveys (LiDAR and photogrammetry) to map canopy height growth and tree mortality over 100 hectares of secondary forest in Indonesia. The timing of the surveys (in 2014, 2017 and 2018) allowed us to assess the impact of a severe El Ni~no Southern Oscillation (ENSO) drought (2015-2016) on forest dynamics and subsequent recovery processes, providing insights into forest resilience to climate change. Early-successional stage forest experienced a 50% loss of canopy height and as much as 90% apparent tree mortality, and taller canopies showed greater proportional resistance to drought. Riparian forests, with greater access to soil water, exhibited less canopy height reduction than forests further away from rivers. In the post-drought period, we observed strong recovery in height growth, particularly in early-successional stands. More established forest had slower recovery and had not consistently returned to pre-drought height two years after the ENSO event. We conclude that recovering forests were resilient to drought lost height was mostly regained within 18 months, suggesting these nature-based solutions may endure as the climate warms.
Keywords: Tropical forest, Restoration, Tree mortality, Resilience, Resistance
Full title: How did the 2015-16 El Niño affect tropical forest restoration in Indonesia?
Abstract: Restoration of logged tropical forests is hailed as a nature-based solution to the interlinked climate and biodiversity crises, but there are concerns that recovery may be hampered by climate change. Here we use repeated airborne surveys (LiDAR and photogrammetry) to map canopy height growth and tree mortality over 100 hectares of secondary forest in Indonesia. The timing of the surveys (in 2014, 2017 and 2018) allowed us to assess the impact of a severe El Ni~no Southern Oscillation (ENSO) drought (2015-2016) on forest dynamics and subsequent recovery processes, providing insights into forest resilience to climate change. Early-successional stage forest experienced a 50% loss of canopy height and as much as 90% apparent tree mortality, and taller canopies showed greater proportional resistance to drought. Riparian forests, with greater access to soil water, exhibited less canopy height reduction than forests further away from rivers. In the post-drought period, we observed strong recovery in height growth, particularly in early-successional stands. More established forest had slower recovery and had not consistently returned to pre-drought height two years after the ENSO event. We conclude that recovering forests were resilient to drought lost height was mostly regained within 18 months, suggesting these nature-based solutions may endure as the climate warms.
Keywords: Tropical forest, Restoration, Tree mortality, Resilience, Resistance
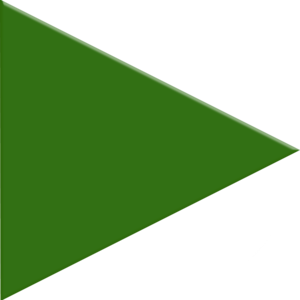
Presented by: Dr. Carsten Montzka, Institute of Bio- and Geosciences: Agrosphere, Forschungszentrum Jülich
Full title: Integration of UAV-based GPR, SAR, LiDAR, Multispectral and Infrared Imaging for Soil and Crop Analysis
Abstract: Carsten Montzka, Kaijun Wu, Jordan Bates, Anke Fluhrer, Maud Henrion, Markus Peichl, Stephan Dill, Philipp Bruecker, Marius Engel, Cosimo Brogi, Thomas Jagdhuber, François Jonard, Sébastien Lambot The presentation introduces a comprehensive experiment that utilizes a fleet of multi-sensor drones to sense agricultural fields in the Terrestrial Environmental Observatories (TERENO) monitoring site Selhausen, Germany. Collaborative efforts from Forschungszentrum Jülich, Université catholique de Louvain, and the German Aerospace Center (DLR) have facilitated the use of a diverse array of sensing technologies. These include a) L-band Synthetic Aperture Radar (SAR) for assessing surface soil moisture and roughness, b) LiDAR for reconstructing topography and measuring crop height, c) low-frequency Ground-Penetrating Radar (GPR) for analyzing root-zone soil electrical conductivity, d) multispectral imaging for evaluating surface soil and crop properties, and e) infrared imaging for monitoring soil surface and crop temperature. Additionally, ground-based multi-configuration electromagnetic induction (EMI) measurements, pedological and topographical maps, along with supplementary satellite imagery, complement the gathered data. Emphasizing the significance of simultaneous multi-sensor imaging and subsequent data integration, the study showcases its effectiveness in capturing diverse properties across various scales. It also highlights observed correlations between different sensor observations, significantly contributing to the field of precision agriculture. The experiment is unique in a holistic interpretation and analysis of soil-vegetation-atmosphere states and exchange processes. However, besides multiple data and corresponding analyses results, the comprehensive measurement campaign opened huge insight to what could be improved and should be optimized for future efforts.
Keywords: Soil, Vegetation, Multi-sensor
Full title: Integration of UAV-based GPR, SAR, LiDAR, Multispectral and Infrared Imaging for Soil and Crop Analysis
Abstract: Carsten Montzka, Kaijun Wu, Jordan Bates, Anke Fluhrer, Maud Henrion, Markus Peichl, Stephan Dill, Philipp Bruecker, Marius Engel, Cosimo Brogi, Thomas Jagdhuber, François Jonard, Sébastien Lambot The presentation introduces a comprehensive experiment that utilizes a fleet of multi-sensor drones to sense agricultural fields in the Terrestrial Environmental Observatories (TERENO) monitoring site Selhausen, Germany. Collaborative efforts from Forschungszentrum Jülich, Université catholique de Louvain, and the German Aerospace Center (DLR) have facilitated the use of a diverse array of sensing technologies. These include a) L-band Synthetic Aperture Radar (SAR) for assessing surface soil moisture and roughness, b) LiDAR for reconstructing topography and measuring crop height, c) low-frequency Ground-Penetrating Radar (GPR) for analyzing root-zone soil electrical conductivity, d) multispectral imaging for evaluating surface soil and crop properties, and e) infrared imaging for monitoring soil surface and crop temperature. Additionally, ground-based multi-configuration electromagnetic induction (EMI) measurements, pedological and topographical maps, along with supplementary satellite imagery, complement the gathered data. Emphasizing the significance of simultaneous multi-sensor imaging and subsequent data integration, the study showcases its effectiveness in capturing diverse properties across various scales. It also highlights observed correlations between different sensor observations, significantly contributing to the field of precision agriculture. The experiment is unique in a holistic interpretation and analysis of soil-vegetation-atmosphere states and exchange processes. However, besides multiple data and corresponding analyses results, the comprehensive measurement campaign opened huge insight to what could be improved and should be optimized for future efforts.
Keywords: Soil, Vegetation, Multi-sensor
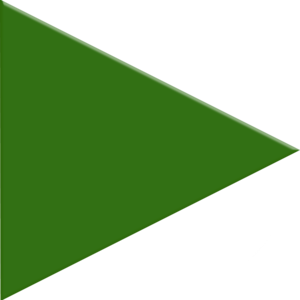
Presented by: Mr Florian Wilken, Augsburg University
Full title: Frequency of rill erosion in the White Nile-Congo ridge region – two years of regular UAV monitoring
Abstract: Tropical Africa is globally one of the most sensitive regions for soil erosion and is characterised by an important yield gap. Rapid population growth is expected to triple food demands in Sub-Saharan Africa by 2050. These rising food demands need to be met by cropland that is highly prone to soil erosion. In particular, the White Nile-Congo ridge region between the DR Congo and Uganda is a hotspot for issues relating to food security linked to massive soil degradation due to steep terrain, highly erosive rainfall and low soil cover conditions. Despite its importance, most soil erosion studies in the region are based on plot or large-scale modelling studies. Both approaches lack information on inter-field connectivity processes, which are especially important in smallholder farming structures where the average field size is 0.1 ha. To address this, a UAV-based monitoring campaign was carried out over different cropland sites (two in the DR Congo and two in Uganda) at high spatial and temporal resolution. The campaign covered more than 500 individual fields which were monitored twice per month (for two years) using UAV-based aerial photography to get insights into event-based rill erosion processes and the role of landscape connectivity. The aerial photography data was classified according to its field condition: (i) vegetation covered, (ii) bare soil without signs of rill erosion, (iii) field with rill erosion. The results highlight the relevance of land cover patchiness due to smallholder farming structures on inter-field connectivity with rill erosion often discontinuing downslope across field boundaries. Therefore, rill development is highly localised and affects individual fields. We further conclude that rill erosion in the White Nile-Congo ridge region is not an episodic process but takes place regularly during the rainy season as a result of high frequency erosive rainfall (on average 20 erosive rainfall events per rainy season) falling on bare soil in fields that are left fallow for individual cultivation periods. Soil erosion dynamics in the study area are complex and controlled by processes that are challenging to be represented in large scale predictions on soil degradation.
Keywords: rill erosion, structural connectivity, event frequency, smallholder farming, wet tropical Africa
Full title: Frequency of rill erosion in the White Nile-Congo ridge region – two years of regular UAV monitoring
Abstract: Tropical Africa is globally one of the most sensitive regions for soil erosion and is characterised by an important yield gap. Rapid population growth is expected to triple food demands in Sub-Saharan Africa by 2050. These rising food demands need to be met by cropland that is highly prone to soil erosion. In particular, the White Nile-Congo ridge region between the DR Congo and Uganda is a hotspot for issues relating to food security linked to massive soil degradation due to steep terrain, highly erosive rainfall and low soil cover conditions. Despite its importance, most soil erosion studies in the region are based on plot or large-scale modelling studies. Both approaches lack information on inter-field connectivity processes, which are especially important in smallholder farming structures where the average field size is 0.1 ha. To address this, a UAV-based monitoring campaign was carried out over different cropland sites (two in the DR Congo and two in Uganda) at high spatial and temporal resolution. The campaign covered more than 500 individual fields which were monitored twice per month (for two years) using UAV-based aerial photography to get insights into event-based rill erosion processes and the role of landscape connectivity. The aerial photography data was classified according to its field condition: (i) vegetation covered, (ii) bare soil without signs of rill erosion, (iii) field with rill erosion. The results highlight the relevance of land cover patchiness due to smallholder farming structures on inter-field connectivity with rill erosion often discontinuing downslope across field boundaries. Therefore, rill development is highly localised and affects individual fields. We further conclude that rill erosion in the White Nile-Congo ridge region is not an episodic process but takes place regularly during the rainy season as a result of high frequency erosive rainfall (on average 20 erosive rainfall events per rainy season) falling on bare soil in fields that are left fallow for individual cultivation periods. Soil erosion dynamics in the study area are complex and controlled by processes that are challenging to be represented in large scale predictions on soil degradation.
Keywords: rill erosion, structural connectivity, event frequency, smallholder farming, wet tropical Africa
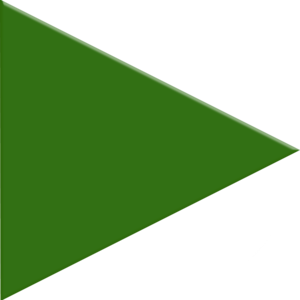
Presented by: Dr Maarten Op de Beeck, University of Antwerp
Full title: Monitoring a pine stand by means of automated drone surveys
Abstract: In an upcoming project, a pine forest near Antwerp (Belgium) will be monitored on a semi-continuous basis by means of automated drone surveys. A drone is being custom build for this purpose and will be equipped with a SIF sensor, a thermal camera and multiple hyperspectral cameras. The drone will carry out repeated, automated flights over the pine stand throughout the entire growing season. The data collected with the drone will be used for quantifying stand productivity, monitoring tree health, and ground truthing of satellite data. The data will also be linked with greenhouse gas flux measurements and canopy reflectance measurements from a fixed eddy covariance tower that is installed in the forest. At the time of writing this abstract, the drone is not yet operational. Test flights are expected to take place in the second half of 2024 and routine flights are expected to start at the beginning of the 2025 growing season.
Keywords: drone, pine forest
Full title: Monitoring a pine stand by means of automated drone surveys
Abstract: In an upcoming project, a pine forest near Antwerp (Belgium) will be monitored on a semi-continuous basis by means of automated drone surveys. A drone is being custom build for this purpose and will be equipped with a SIF sensor, a thermal camera and multiple hyperspectral cameras. The drone will carry out repeated, automated flights over the pine stand throughout the entire growing season. The data collected with the drone will be used for quantifying stand productivity, monitoring tree health, and ground truthing of satellite data. The data will also be linked with greenhouse gas flux measurements and canopy reflectance measurements from a fixed eddy covariance tower that is installed in the forest. At the time of writing this abstract, the drone is not yet operational. Test flights are expected to take place in the second half of 2024 and routine flights are expected to start at the beginning of the 2025 growing season.
Keywords: drone, pine forest
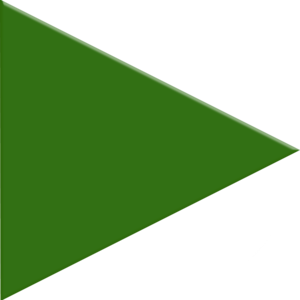
Presented by: Mr Kim-Cedric Gröschler, Kiel University
Full title: Monitoring the Population Development of Indicator Plants in High Nature Value Grassland Using Machine Learning and Drone Data
Abstract: Monitoring indicator plant species in high nature value grasslands is vital for nature conservation, but traditional methods are resource-intensive. In a recent study, we showcased the efficacy of repeated drone-based plant counts for monitoring the population development of Dactylorhiza majalis (DM). Utilizing multispectral drone data over two consecutive flowering seasons, we employed Random Forest and Neural Network approaches for remote sensing plant counts (RSPC). Compared to in situ data, Random Forest-based RSPC outperformed Neural Network-based RSPC, achieving an R² of 0.8 and 0.63, with RMSE of 8.5 and 11.4 DM individuals/m² respectively. The accuracies indicate a comparable performance to conventional plant count surveys. Change detection revealed an overall decline in DM individuals in our study site, with limited increases observed in specific regions. With our approach, we were able to document the success of manual DM seed transfers to a previously uninhabited area within our study site, highlighting the potential of drone surveys for monitoring and aiding conservation efforts.
Keywords: remote sensing, uav, nature conservation, grassland, machine learning
Full title: Monitoring the Population Development of Indicator Plants in High Nature Value Grassland Using Machine Learning and Drone Data
Abstract: Monitoring indicator plant species in high nature value grasslands is vital for nature conservation, but traditional methods are resource-intensive. In a recent study, we showcased the efficacy of repeated drone-based plant counts for monitoring the population development of Dactylorhiza majalis (DM). Utilizing multispectral drone data over two consecutive flowering seasons, we employed Random Forest and Neural Network approaches for remote sensing plant counts (RSPC). Compared to in situ data, Random Forest-based RSPC outperformed Neural Network-based RSPC, achieving an R² of 0.8 and 0.63, with RMSE of 8.5 and 11.4 DM individuals/m² respectively. The accuracies indicate a comparable performance to conventional plant count surveys. Change detection revealed an overall decline in DM individuals in our study site, with limited increases observed in specific regions. With our approach, we were able to document the success of manual DM seed transfers to a previously uninhabited area within our study site, highlighting the potential of drone surveys for monitoring and aiding conservation efforts.
Keywords: remote sensing, uav, nature conservation, grassland, machine learning
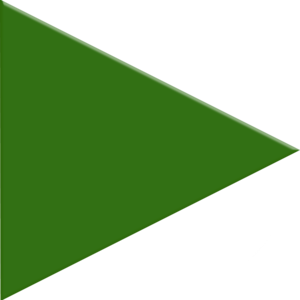
Presented by: PhD student Saja Salattna, Institute for Bio- and Geosciences Plant Sciences (IBG-2)
Full title: Imaging very high spatial resolution solar-induced chlorophyll fluorescence (SIF) from an uncrewed aerial vehicle (UAS)
Abstract: Remote sensing of solar-induced chlorophyll fluorescence (SIF) is rapidly developing to track vegetation's photosynthetic activity and related stress from the leaf to the ecosystem level at scales ranging from in-situ measurements to satellite products. Airborne sensors, such as HyPlant, are promising tools for measuring the SIF of larger areas. However, airborne data have limited spatial (1-4 m pixel size), and data acquisition is relatively expensive. The main objective of this study is to produce a centimeter-resolution SIF map of an agriculture field using the SIFcam, a small-scale snapshot dual-camera system mounted on an uncrewed aerial system (UAS). SIFcam is based on optical interference filters that enable measuring SIF at 760 nm with a pixel size of 0.04 m when flown 70 m above the ground level. The presented study gives a detailed overview of the processing chain for the SIFcam dual-camera system and the processing steps consisting of raw data preprocessing (flat field and dark current correction), radiometric correction, top-of-canopy reflectance calculation, orthomosaic generation, and retrieval of SIF at 760 nm using the Fraunhofer Line Depth (FLD) method. Two distinct processing workflows were outlined. Subsequently, a sensitivity analysis was conducted to assess the accuracy of these workflows; SIF data is evaluated against observations obtained from the mobile ground-based non-imaging system FloX and imagery acquired by the HyPlant airborne sensor. Additionally, we present a case study of SIF retrieval to evaluate the potential of the system for understanding diurnal SIF patterns.
Keyword: camera-based SIF measurement, photosynthetic dynamics, remote sensing of vegetation, solar-induced c
Full title: Imaging very high spatial resolution solar-induced chlorophyll fluorescence (SIF) from an uncrewed aerial vehicle (UAS)
Abstract: Remote sensing of solar-induced chlorophyll fluorescence (SIF) is rapidly developing to track vegetation's photosynthetic activity and related stress from the leaf to the ecosystem level at scales ranging from in-situ measurements to satellite products. Airborne sensors, such as HyPlant, are promising tools for measuring the SIF of larger areas. However, airborne data have limited spatial (1-4 m pixel size), and data acquisition is relatively expensive. The main objective of this study is to produce a centimeter-resolution SIF map of an agriculture field using the SIFcam, a small-scale snapshot dual-camera system mounted on an uncrewed aerial system (UAS). SIFcam is based on optical interference filters that enable measuring SIF at 760 nm with a pixel size of 0.04 m when flown 70 m above the ground level. The presented study gives a detailed overview of the processing chain for the SIFcam dual-camera system and the processing steps consisting of raw data preprocessing (flat field and dark current correction), radiometric correction, top-of-canopy reflectance calculation, orthomosaic generation, and retrieval of SIF at 760 nm using the Fraunhofer Line Depth (FLD) method. Two distinct processing workflows were outlined. Subsequently, a sensitivity analysis was conducted to assess the accuracy of these workflows; SIF data is evaluated against observations obtained from the mobile ground-based non-imaging system FloX and imagery acquired by the HyPlant airborne sensor. Additionally, we present a case study of SIF retrieval to evaluate the potential of the system for understanding diurnal SIF patterns.
Keyword: camera-based SIF measurement, photosynthetic dynamics, remote sensing of vegetation, solar-induced c
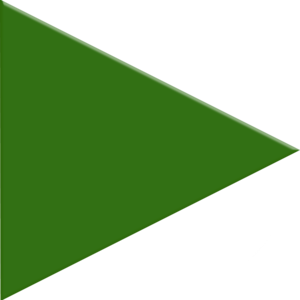
Presented by: Mr Dennis Löwe, Julius Kühn-Institut (JKI)
Full title: UAV-based Phenotyping of Oilseed Rape Hybrids Using Multivariate Statistics and Machine Learning Methods
Abstract: Global changes in climate, economic conditions, and population growth exert substantial pressure on agricultural practices, making synthetic fertilizers, particularly nitrogen-based fertilizers, essential to agricultural productivity. In 2018, nitrogen fertilizers constituted 58% of total fertilizer usage. However, their production is energy-intensive, and their application poses environmental risks such as groundwater contamination and the release of nitrous oxide (N₂O), a major greenhouse gas. Our aim is to mitigate these environmental impacts by employing digital phenotyping in field crop breeding to develop nitrogen-efficient winter oilseed rape (WOSR) hybrids. This approach seeks to reduce greenhouse gas emissions and enhance resource use efficiency. To achieve these goals, we focuses on developing UAV-based (Unmanned Aerial Vehicles) models that facilitate high-throughput testing of numerous winter oilseed rape phenotypes to identify nitrogen efficient genotypes. A nitrogen enhancement trial was conducted at the Julius Kühn Institute in Quedlinburg, involving five genotypes, five nitrogen fertilizer levels, and 80 plots. During the vegetation period, drone-based multispectral imaging (MicaSense DualMX) was employed to capture high-resolution temporal image data. Additional UAV data were collected from four other plant-breeding sites in northern Germany. This data collection was complemented by in-situ sampling to develop initial predictive models for fresh biomass and plant nitrogen content in WOSR. All work was carried out as part of the NORA project funded by the BMEL. Results from the first year of field trials indicated that Random Forest and Partial Least Squares Regression were particularly effective in modeling the target variables, whereas Support Vector Machine models demonstrated the lowest predictive accuracy. In addition to the initial findings and optimized models, this poster will present results on the resource use efficiency of the tested hybrid WOSR varieties.
Keywords: multispectral, crop phenotyping, machine learning, winter oilseed rape
Full title: UAV-based Phenotyping of Oilseed Rape Hybrids Using Multivariate Statistics and Machine Learning Methods
Abstract: Global changes in climate, economic conditions, and population growth exert substantial pressure on agricultural practices, making synthetic fertilizers, particularly nitrogen-based fertilizers, essential to agricultural productivity. In 2018, nitrogen fertilizers constituted 58% of total fertilizer usage. However, their production is energy-intensive, and their application poses environmental risks such as groundwater contamination and the release of nitrous oxide (N₂O), a major greenhouse gas. Our aim is to mitigate these environmental impacts by employing digital phenotyping in field crop breeding to develop nitrogen-efficient winter oilseed rape (WOSR) hybrids. This approach seeks to reduce greenhouse gas emissions and enhance resource use efficiency. To achieve these goals, we focuses on developing UAV-based (Unmanned Aerial Vehicles) models that facilitate high-throughput testing of numerous winter oilseed rape phenotypes to identify nitrogen efficient genotypes. A nitrogen enhancement trial was conducted at the Julius Kühn Institute in Quedlinburg, involving five genotypes, five nitrogen fertilizer levels, and 80 plots. During the vegetation period, drone-based multispectral imaging (MicaSense DualMX) was employed to capture high-resolution temporal image data. Additional UAV data were collected from four other plant-breeding sites in northern Germany. This data collection was complemented by in-situ sampling to develop initial predictive models for fresh biomass and plant nitrogen content in WOSR. All work was carried out as part of the NORA project funded by the BMEL. Results from the first year of field trials indicated that Random Forest and Partial Least Squares Regression were particularly effective in modeling the target variables, whereas Support Vector Machine models demonstrated the lowest predictive accuracy. In addition to the initial findings and optimized models, this poster will present results on the resource use efficiency of the tested hybrid WOSR varieties.
Keywords: multispectral, crop phenotyping, machine learning, winter oilseed rape
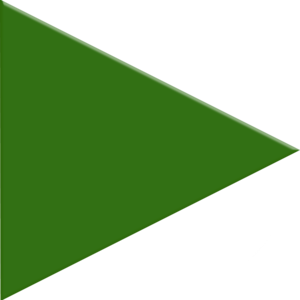
Presented by: Dr Rachael Thornley, University of Oxford
Full title: Using high spatial resolution images to monitor grassland plant resilience
Abstract: The biodiversity and ecological functioning of grassland systems are under threat from suboptimal management, land conversion, and nutrient addition. Community level responses to these disturbances are well documented, but the mechanisms that lead to such responses are less well understood. Demographic modelling of grassland plant populations could provide key insights. However, one challenge in developing these models is that they require large data sets, with repeat observations, often impossible to capture at scale using traditional methods. High-resolution RGB and hyperspectral images, captured by UAVs and pole mounted cameras, offer an opportunity for fast, reliable monitoring of herbaceous communities and the tracking of individual plants over time. Exploitation of morphological information, alongside leaf and canopy level spectral reflectance, may enable important qualitative and quantitative information to be gathered. However, grassland canopies present challenges for image analysis due to their complex structure and the often-high turnover of individuals within these ecosystems. Here, we present preliminary results regarding the characterisation of UK grasslands. Images of grassland quadrats were captured at high spatial resolution and segmented to three levels of complexity: functional group, taxonomic group, and individuals. We demonstrate the application of these methods to plant population ecology, where the monitoring of individual plant performance (e.g., survival, growth, reproduction) is a key input into data hungry demographic models.
Keywords: grasslands, image analysis, demography, high-resolution
Full title: Using high spatial resolution images to monitor grassland plant resilience
Abstract: The biodiversity and ecological functioning of grassland systems are under threat from suboptimal management, land conversion, and nutrient addition. Community level responses to these disturbances are well documented, but the mechanisms that lead to such responses are less well understood. Demographic modelling of grassland plant populations could provide key insights. However, one challenge in developing these models is that they require large data sets, with repeat observations, often impossible to capture at scale using traditional methods. High-resolution RGB and hyperspectral images, captured by UAVs and pole mounted cameras, offer an opportunity for fast, reliable monitoring of herbaceous communities and the tracking of individual plants over time. Exploitation of morphological information, alongside leaf and canopy level spectral reflectance, may enable important qualitative and quantitative information to be gathered. However, grassland canopies present challenges for image analysis due to their complex structure and the often-high turnover of individuals within these ecosystems. Here, we present preliminary results regarding the characterisation of UK grasslands. Images of grassland quadrats were captured at high spatial resolution and segmented to three levels of complexity: functional group, taxonomic group, and individuals. We demonstrate the application of these methods to plant population ecology, where the monitoring of individual plant performance (e.g., survival, growth, reproduction) is a key input into data hungry demographic models.
Keywords: grasslands, image analysis, demography, high-resolution
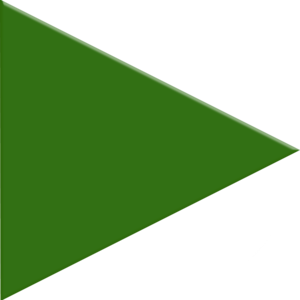
Presented by: Senior Research Scientist Melina Zempila, RAL Space
Full title: HyperSpectral Imaging for UAS-based wheat crop monitoring
Abstract: RAL Space has developed a hyper-spectral imaging (HSI) system that focuses on the spectral window 470-920 nm and meets the demands of static sampling and remote sensing when mounted on an Unmanned Aerial System (UAS). The system has been already deployed in several in house studies: detection of dothistroma in Scots pine needles, starch detection in apples and bananas, and avocados maturity indication, while aerial imagery was also acquired during field campaigns in the UK and China aiming to create a tree species distribution map and to early identify tree health issues. Lately the system has been successfully deployed in the field to early detect yellow and brown rust in winter wheat, whereas flights at three different heights were performed over wheat plots of different Nitrogen treatment to assess the impact of increasing separation distance at Nitrogen uptake efficiency remote sensing techniques.
Keywords: UAS, Hyperspectral imaging, Remote Sensing, Classification, Calibration
Full title: HyperSpectral Imaging for UAS-based wheat crop monitoring
Abstract: RAL Space has developed a hyper-spectral imaging (HSI) system that focuses on the spectral window 470-920 nm and meets the demands of static sampling and remote sensing when mounted on an Unmanned Aerial System (UAS). The system has been already deployed in several in house studies: detection of dothistroma in Scots pine needles, starch detection in apples and bananas, and avocados maturity indication, while aerial imagery was also acquired during field campaigns in the UK and China aiming to create a tree species distribution map and to early identify tree health issues. Lately the system has been successfully deployed in the field to early detect yellow and brown rust in winter wheat, whereas flights at three different heights were performed over wheat plots of different Nitrogen treatment to assess the impact of increasing separation distance at Nitrogen uptake efficiency remote sensing techniques.
Keywords: UAS, Hyperspectral imaging, Remote Sensing, Classification, Calibration
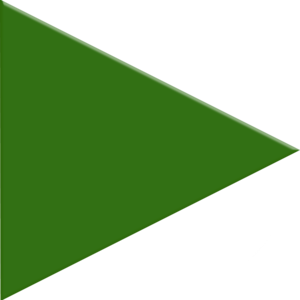
Presented by: Mr. Noah Mihatsch, BOKU University
Full title: Multitemporal and Multispectral Drone Data for Classifying Tree Species in an Austrian Riparian Forest
Abstract: The climate crisis is threatening native forests all over Europe and a change in the composition of species can be expected in the future. Diversity of tree species is one key aspect of resilience against the climatic stress caused by the climate crisis. To maintain one of the last huge floodplains in Europe – the Danube Floodplain – for future generations, it is necessary to monitor the change of the species distribution and the development of eco-systems. Remote sensing is widely used for establishing a constant monitoring of forests, including tree species classification (TSC). Currently, Unmanned Aerial Vehicles (UAVs) offer very high-resolution data together with temporal flexibility and cost efficiency which can be used in the management practice of forests and national parks in particular. However, due to the extensive diversity inherent in different forest types and tree species, the results obtained in the state-of-art research in TSC via very high-resolution optical data cannot be generalised. As there is still a gap in research in the field of TSC in riparian forests, this study aims at filling this gap with preliminary results of TSC in a riparian forest, namely the Danube Floodplain National Park (Austria). Therefore, three drone flights were conducted during October, September, and May spanning the years 2021 and 2022 together with a simultaneous collection of reference data in the field. Tree crowns were delineated manually in two different ways: point-buffered and exact delineation of the crown shape. Multiple object-based Random Forest models were performed, comparing mono- and multitemporal data as well as two different spatial resolutions (3.0 cm and 6.4 cm) and the two different levels of detail of the delineation of tree crowns. Highest Overall Accuracy (OA) for 12 different tree species and one dead wood class could be reached by the multitemporal model at 82.1 % (kappa = 80.8 %) with the higher spatial resolution (3.0 cm) and the exact delineation of the reference data. Producer’s Accuracy (PA) and User’s Accuracy (UA) varied between 50 % and 100 % for different classes. (As no significant difference in the OA was found between the two spatial resolutions, higher flight heights can be used to cover more area). Promising results from this study showed that the presented method can be used for precise monitoring of tree species diversity in the Danube Floodplain National Park. Further improvement could be reached by merging data from different sensors.
Full title: Multitemporal and Multispectral Drone Data for Classifying Tree Species in an Austrian Riparian Forest
Abstract: The climate crisis is threatening native forests all over Europe and a change in the composition of species can be expected in the future. Diversity of tree species is one key aspect of resilience against the climatic stress caused by the climate crisis. To maintain one of the last huge floodplains in Europe – the Danube Floodplain – for future generations, it is necessary to monitor the change of the species distribution and the development of eco-systems. Remote sensing is widely used for establishing a constant monitoring of forests, including tree species classification (TSC). Currently, Unmanned Aerial Vehicles (UAVs) offer very high-resolution data together with temporal flexibility and cost efficiency which can be used in the management practice of forests and national parks in particular. However, due to the extensive diversity inherent in different forest types and tree species, the results obtained in the state-of-art research in TSC via very high-resolution optical data cannot be generalised. As there is still a gap in research in the field of TSC in riparian forests, this study aims at filling this gap with preliminary results of TSC in a riparian forest, namely the Danube Floodplain National Park (Austria). Therefore, three drone flights were conducted during October, September, and May spanning the years 2021 and 2022 together with a simultaneous collection of reference data in the field. Tree crowns were delineated manually in two different ways: point-buffered and exact delineation of the crown shape. Multiple object-based Random Forest models were performed, comparing mono- and multitemporal data as well as two different spatial resolutions (3.0 cm and 6.4 cm) and the two different levels of detail of the delineation of tree crowns. Highest Overall Accuracy (OA) for 12 different tree species and one dead wood class could be reached by the multitemporal model at 82.1 % (kappa = 80.8 %) with the higher spatial resolution (3.0 cm) and the exact delineation of the reference data. Producer’s Accuracy (PA) and User’s Accuracy (UA) varied between 50 % and 100 % for different classes. (As no significant difference in the OA was found between the two spatial resolutions, higher flight heights can be used to cover more area). Promising results from this study showed that the presented method can be used for precise monitoring of tree species diversity in the Danube Floodplain National Park. Further improvement could be reached by merging data from different sensors.
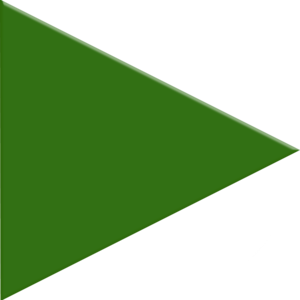
Presented by: Dr. Daniel Rutte, Deutsches Zentrum für Schienenverkehrsforschung beim Eisenbahn-Bundesamt
Full title: Investigating tree vitality along railways – UAV data in a multi-method approach
Abstract: Tree vitality is a driving factor of natural hazard-related risks for rail transport, but has so far been given little consideration in risk models and management concepts. This is mainly due to lack of reliable tree vitality data along railways. In the commencing mFUND financed project RailVitaliTree – Tree vitality monitoring and modeling of drought-related risks along railroads with remote sensing and dendroecology, we develop a tree vitality monitor for the tree population along the german rail network. The project aims to use the analysis of time-series data (multispectral satellite, dendroecology and climate data) to improve our understanding of the climate-vitality relationship in trees in the specific microclimate along railways. We will use UAV-based multispectral and LiDAR sensors to investigate training sites to aid the analysis of nationwide multispectral satellite imagery. Based on our findings, we will evaluate the long-term consequences of drought and its multiplier effects for other natural hazard-related risks in order to increase the resilience of rail transport to vegetation-related disturbances. Here, we present our first steps, study sites and methodology with a focus on UAV-based analysis of vegetation.
Keywords: Tree vitality, natural hazard, rail
Full title: Investigating tree vitality along railways – UAV data in a multi-method approach
Abstract: Tree vitality is a driving factor of natural hazard-related risks for rail transport, but has so far been given little consideration in risk models and management concepts. This is mainly due to lack of reliable tree vitality data along railways. In the commencing mFUND financed project RailVitaliTree – Tree vitality monitoring and modeling of drought-related risks along railroads with remote sensing and dendroecology, we develop a tree vitality monitor for the tree population along the german rail network. The project aims to use the analysis of time-series data (multispectral satellite, dendroecology and climate data) to improve our understanding of the climate-vitality relationship in trees in the specific microclimate along railways. We will use UAV-based multispectral and LiDAR sensors to investigate training sites to aid the analysis of nationwide multispectral satellite imagery. Based on our findings, we will evaluate the long-term consequences of drought and its multiplier effects for other natural hazard-related risks in order to increase the resilience of rail transport to vegetation-related disturbances. Here, we present our first steps, study sites and methodology with a focus on UAV-based analysis of vegetation.
Keywords: Tree vitality, natural hazard, rail
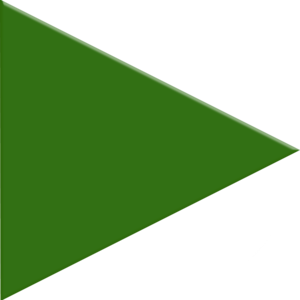
Presented by: Mr Timon Boos, Agroscope, University of Zürich
Full title: Using UAVs to estimate canopy cover with high resolution satellite data
Abstract: Traditional methods for observing crop traits are often time-consuming and limited in spatial and temporal resolution. Satellite- and UAV-based remote sensing offer a non-destructive and efficient way to systematically gather phenological data. The advent of multispectral Super-Dove CubeSats from PlanetLabs has addressed the limitations of traditional satellite platforms by significantly improving the spatial and temporal resolution of satellite data. Still, there remains uncertainty regarding the spectral and spatial precision of this satellite imagery. Furthermore, the Leaf Area Index (LAI), a commonly used metric for quantifying plant growth, has been found to exhibit limitations in the initial phase of plant development. To address these challenges, we employ high-resolution UAV data to calibrate and validate satellite imagery with in-situ canopy cover information. Initially, canopy cover of various crops is extracted using high-resolution RGB imagery from UAVs. The extracted canopy cover data is then compared with multispectral data from PlanetLabs to identify a representative vegetation index (VI) for the early growth stage. This approach enhances our understanding of the accuracy of high-resolution satellite data and its applicability for biomass monitoring.
Keywords: remote sensing, planet labs, high-resolution, UAV, canopy cover
Full title: Using UAVs to estimate canopy cover with high resolution satellite data
Abstract: Traditional methods for observing crop traits are often time-consuming and limited in spatial and temporal resolution. Satellite- and UAV-based remote sensing offer a non-destructive and efficient way to systematically gather phenological data. The advent of multispectral Super-Dove CubeSats from PlanetLabs has addressed the limitations of traditional satellite platforms by significantly improving the spatial and temporal resolution of satellite data. Still, there remains uncertainty regarding the spectral and spatial precision of this satellite imagery. Furthermore, the Leaf Area Index (LAI), a commonly used metric for quantifying plant growth, has been found to exhibit limitations in the initial phase of plant development. To address these challenges, we employ high-resolution UAV data to calibrate and validate satellite imagery with in-situ canopy cover information. Initially, canopy cover of various crops is extracted using high-resolution RGB imagery from UAVs. The extracted canopy cover data is then compared with multispectral data from PlanetLabs to identify a representative vegetation index (VI) for the early growth stage. This approach enhances our understanding of the accuracy of high-resolution satellite data and its applicability for biomass monitoring.
Keywords: remote sensing, planet labs, high-resolution, UAV, canopy cover
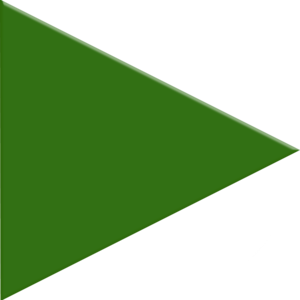
Presented by: Ms Malin Stephan, University of Greifswald, Institute for Geography and Geology
Full title: Towards an intra-annual monitoring of peatland vegetation composition by spatially and temporally transferable classification models
Abstract: Peatlands are important ecosystems, e.g. in the context of biodiversity conservation but even more for mitigating climate change. One aspect is their ability to store carbon under wet conditions, in the form of dead plant material. Many peatlands have been drained especially for agricultural purposes, resulting in high GHG emissions. Thus, drained peatlands need to get rewetted. Regular monitoring concepts are necessary in order to evaluate rewetting measures. The composition of the vegetation cover can give an indication on wetness, but vegetation structures may be heterogeneous and complex throughout the vegetation period. Intra-specific differences in phenology and intra-annual changes in water level are challenging in classifying peatland vegetation. UAV data can be acquired on a regular basis and have a sufficiently high spatial resolution to monitor the impact of changing water levels on the vegetation development. In order to operationalize and standardize such monitoring we here test the transferability of classification models through space and time. Our study site is a rewetted fen peatland in Peene river valley, NE Germany. We have acquired UAV data on 3 dates in 2023 (06 June, 22 August and 07 September) with a multispectral Micasense Altum camera on a DJI Matrice 300. In addition, we used a normalized digital surface model (nDSM) from a DJI L1 sensor, normalized with a 1 m digital terrain model from state authorities. Data were preprocessed, co-registered and resampled to 50 cm. For these aggregated pixels different metrics (mean, StDev, min and max for the multispectral image and nine percentiles from 10th to 90th for the nDSM) were derived. We then classified peatland vegetation, e.g., Phragmites australis, Carex spp. or Phalaris arundinacea, using a Random Forest classifier. Reference pixels were selected based on field notes and then split in training and validation data sets (70/30). First models were successfully trained using spectral information from the southern half of the study site in August together with the aggregated height information and both the elevation data and aggregation proved useful for the map accuracy. As a next step, different variations of these models will be compared during model transfer and evaluated with vegetation inventory data. The vegetation inventory data were recorded close to the UAV flights. The present results will be used to validate quantitative vegetation class fraction maps from spaceborne EnMAP data. When results for multiple dates are available, especially the differences in water table and observable plant cover will be better understand during the larger scale analysis.
Keywords: peatlands, monitoring, model transfer, multispectral, digital surface model
Poster Dataset: Poster PDF
Full title: Towards an intra-annual monitoring of peatland vegetation composition by spatially and temporally transferable classification models
Abstract: Peatlands are important ecosystems, e.g. in the context of biodiversity conservation but even more for mitigating climate change. One aspect is their ability to store carbon under wet conditions, in the form of dead plant material. Many peatlands have been drained especially for agricultural purposes, resulting in high GHG emissions. Thus, drained peatlands need to get rewetted. Regular monitoring concepts are necessary in order to evaluate rewetting measures. The composition of the vegetation cover can give an indication on wetness, but vegetation structures may be heterogeneous and complex throughout the vegetation period. Intra-specific differences in phenology and intra-annual changes in water level are challenging in classifying peatland vegetation. UAV data can be acquired on a regular basis and have a sufficiently high spatial resolution to monitor the impact of changing water levels on the vegetation development. In order to operationalize and standardize such monitoring we here test the transferability of classification models through space and time. Our study site is a rewetted fen peatland in Peene river valley, NE Germany. We have acquired UAV data on 3 dates in 2023 (06 June, 22 August and 07 September) with a multispectral Micasense Altum camera on a DJI Matrice 300. In addition, we used a normalized digital surface model (nDSM) from a DJI L1 sensor, normalized with a 1 m digital terrain model from state authorities. Data were preprocessed, co-registered and resampled to 50 cm. For these aggregated pixels different metrics (mean, StDev, min and max for the multispectral image and nine percentiles from 10th to 90th for the nDSM) were derived. We then classified peatland vegetation, e.g., Phragmites australis, Carex spp. or Phalaris arundinacea, using a Random Forest classifier. Reference pixels were selected based on field notes and then split in training and validation data sets (70/30). First models were successfully trained using spectral information from the southern half of the study site in August together with the aggregated height information and both the elevation data and aggregation proved useful for the map accuracy. As a next step, different variations of these models will be compared during model transfer and evaluated with vegetation inventory data. The vegetation inventory data were recorded close to the UAV flights. The present results will be used to validate quantitative vegetation class fraction maps from spaceborne EnMAP data. When results for multiple dates are available, especially the differences in water table and observable plant cover will be better understand during the larger scale analysis.
Keywords: peatlands, monitoring, model transfer, multispectral, digital surface model
Poster Dataset: Poster PDF
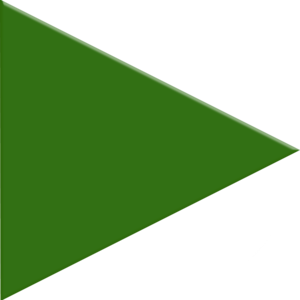
Presented by: Ms Eva Wickert, Institute of Natural Hazards and Georisks, RWTH Aachen University
Full title: Investigating lichen occurrence on volcanic material with UAV-based hyperspectral remote sensing
Abstract: As one of the first organisms to establish on cooled lava flows, lichens can indicate valuable information about the volcanic terrain such as age, weathering state or gas emissions. Their individual spectral signatures can be used to distinguish between different groups of lichens (e.g. crustose and fruticose), allowing conclusions to be drawn about, for instance, different intensities of weathering processes and demonstrating their spatial and temporal dimensions. We combine data collected from a hyperspectral UAV survey and field spectrometer measurements from lichens on volcanic material at Fagradalsfjall volcano in Iceland to analyze the characteristics of the spectral signatures as a foundation for assessing spatial distribution patterns. Therefore, we propose an open-source and Python-based (post-)processing workflow that integrates the different datasets and allows them to complement each other to improve the analysis. Our goal is to investigate the relationship between distribution patterns and substrate properties to trace the processes of lava flow emplacement and subsequent geochemical processes. We emphasize the necessity to expand spectral signature databases and improve sampling methods. These enhancements will not only benefit our research but also support advancements in research projects across various disciplines. Finally, we suggest incorporating data from satellite-based hyperspectral missions (e.g., EnMAP) into this workflow to facilitate large-scale mapping of lichens in volcanic terrain.
Full title: Investigating lichen occurrence on volcanic material with UAV-based hyperspectral remote sensing
Abstract: As one of the first organisms to establish on cooled lava flows, lichens can indicate valuable information about the volcanic terrain such as age, weathering state or gas emissions. Their individual spectral signatures can be used to distinguish between different groups of lichens (e.g. crustose and fruticose), allowing conclusions to be drawn about, for instance, different intensities of weathering processes and demonstrating their spatial and temporal dimensions. We combine data collected from a hyperspectral UAV survey and field spectrometer measurements from lichens on volcanic material at Fagradalsfjall volcano in Iceland to analyze the characteristics of the spectral signatures as a foundation for assessing spatial distribution patterns. Therefore, we propose an open-source and Python-based (post-)processing workflow that integrates the different datasets and allows them to complement each other to improve the analysis. Our goal is to investigate the relationship between distribution patterns and substrate properties to trace the processes of lava flow emplacement and subsequent geochemical processes. We emphasize the necessity to expand spectral signature databases and improve sampling methods. These enhancements will not only benefit our research but also support advancements in research projects across various disciplines. Finally, we suggest incorporating data from satellite-based hyperspectral missions (e.g., EnMAP) into this workflow to facilitate large-scale mapping of lichens in volcanic terrain.
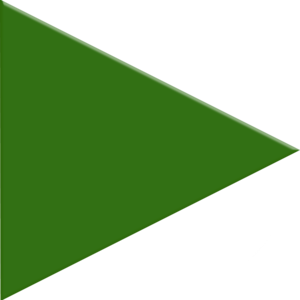
Presented by: M Sc Christina Hellmann, University Greifswald, Insitute for Geography and Geology
Full title: Improving hyperspectral monitoring of peatland vegetation: Combining field spectroscopy, airborne and spaceborne imaging spectroscopy
Abstract: Drained peatlands are carbon sources contributing 4% to global greenhouse gas emissions. Rewetting of drained peatlands becomes crucial to mitigate carbon emissions. Concepts are required to monitor rewetting measures. Vegetation, for example, can give an indication on the underlying gradients, such as wetness or nutrient conditions. Current hyperspectral spaceborne missions (e.g., EnMAP) offer high spectral resolution data for relatively large areas with ideally multiple acquisitions per year, yet, low spatial resolution (30m). Since vegetation patterns can be heterogeneous, we want to map peatland vegetation quantitively at subpixel scale from EnMAP imagery. Supported by ASD field spectra and hyperspectral UAV data we want to answer the two following questions: (1) Can the additional information from HS UAV data further improve multispectral classification results? (2) Can hyperspectral UAV data contribute to reconstructing spectrally mixed EnMAP pixels from field spectra. By answering these two questions we hope to understand which peatland species can be differentiated from space. The study area is located in NE-Germany and was rewetted in 2000. We sampled ASD field measurements (full range) from the main dominant species throughout the vegetation periods in 2023 and 2024. The study area is covered by three EnMAP acquisitions, June and August 2023 and May 2024. Additional acquisitions from summer 2024 will be included. The hyperspectral UAV (VISNIR) surveys were undertaken in June 2023 and May 2024. For the first research question, monthly classifications for the two years and the main dominant species (e.g., Phragmites australis, Phalaris arundinacea, Carex spp.) from multispectral UAV data (see Stephan et al. 2024, this workshop), will be used for comparison to hyperspectral classification results. We want to answer the second research question by comparing the variation within the ASD measurements and the hyperspectral drone imagery for the main target species. Using a forward mixing approach, class wise field spectra were weighted according to their respective spatial extent from the classification results to build a set of synthetic EnMAP pixels after spectral resampling. This synthetic EnMAP pixel can then be compared to the real pixel. Results from backwards unmixing of multiple of such EnMAP pixel pairs, each representing a real land surface composition, will help us to identify quantifiable peatland species from space. With ongoing and new upcoming spaceborne missions (PRISMA, SBG, CHIME), UAV data with high spatial resolution and preferably high spectral resolution, will be crucial in understanding spaceborne imagery. We hope with this study to bring a large-scale monitoring workflow assessing upcoming rewetting efforts, forward.
Keywords: peatland vegetation mapping, spatial scale, EnMAP, UAV
Full title: Improving hyperspectral monitoring of peatland vegetation: Combining field spectroscopy, airborne and spaceborne imaging spectroscopy
Abstract: Drained peatlands are carbon sources contributing 4% to global greenhouse gas emissions. Rewetting of drained peatlands becomes crucial to mitigate carbon emissions. Concepts are required to monitor rewetting measures. Vegetation, for example, can give an indication on the underlying gradients, such as wetness or nutrient conditions. Current hyperspectral spaceborne missions (e.g., EnMAP) offer high spectral resolution data for relatively large areas with ideally multiple acquisitions per year, yet, low spatial resolution (30m). Since vegetation patterns can be heterogeneous, we want to map peatland vegetation quantitively at subpixel scale from EnMAP imagery. Supported by ASD field spectra and hyperspectral UAV data we want to answer the two following questions: (1) Can the additional information from HS UAV data further improve multispectral classification results? (2) Can hyperspectral UAV data contribute to reconstructing spectrally mixed EnMAP pixels from field spectra. By answering these two questions we hope to understand which peatland species can be differentiated from space. The study area is located in NE-Germany and was rewetted in 2000. We sampled ASD field measurements (full range) from the main dominant species throughout the vegetation periods in 2023 and 2024. The study area is covered by three EnMAP acquisitions, June and August 2023 and May 2024. Additional acquisitions from summer 2024 will be included. The hyperspectral UAV (VISNIR) surveys were undertaken in June 2023 and May 2024. For the first research question, monthly classifications for the two years and the main dominant species (e.g., Phragmites australis, Phalaris arundinacea, Carex spp.) from multispectral UAV data (see Stephan et al. 2024, this workshop), will be used for comparison to hyperspectral classification results. We want to answer the second research question by comparing the variation within the ASD measurements and the hyperspectral drone imagery for the main target species. Using a forward mixing approach, class wise field spectra were weighted according to their respective spatial extent from the classification results to build a set of synthetic EnMAP pixels after spectral resampling. This synthetic EnMAP pixel can then be compared to the real pixel. Results from backwards unmixing of multiple of such EnMAP pixel pairs, each representing a real land surface composition, will help us to identify quantifiable peatland species from space. With ongoing and new upcoming spaceborne missions (PRISMA, SBG, CHIME), UAV data with high spatial resolution and preferably high spectral resolution, will be crucial in understanding spaceborne imagery. We hope with this study to bring a large-scale monitoring workflow assessing upcoming rewetting efforts, forward.
Keywords: peatland vegetation mapping, spatial scale, EnMAP, UAV
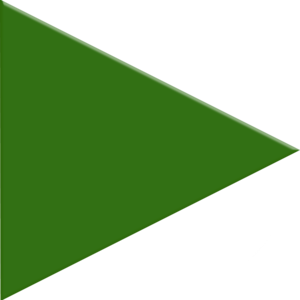
Presented by: Mr Richard Georgi, OGF GmbH
Full title: Determining Wildlife Abundance Using UAVs – Practical Experiences
Abstract: Accurate estimation of wildlife abundance is crucial for effective wildlife management and understanding their impact on vegetation and biodiversity. The use of Unmanned Aerial Vehicles (UAVs, drones) allows for precise monitoring of numerous wildlife species, though species and habitat-specific differences exist. Based on aerial surveys covering over 100,000 hectares using DJI M300 RTK and DJI M30T drones, practical experiences in UAV-based wildlife detection are presented, highlighting the influence of wildlife on vegetation patterns. Wildlife species react differently to UAVs; wild boars and roe deer rarely respond to drones flying at altitudes around and above 70 meters, whereas red deer and predators often detect UAVs and may exhibit flight behavior. Modern drones, given suitable conditions, can reliably detect and identify many wildlife species from over 100 meters away. The high-resolution optics of drones also provide additional information on gender and age distribution when needed. Drones enable GPS-accurate localization of animals, and combining this spatial distribution data with information on forest structure, hunting regimes, and vegetation patterns can yield many insightful correlations. Understanding these interactions helps in assessing the impact of wildlife on vegetation and overall biodiversity. The daily coverage area with the mentioned models ranges from approximately 300 to over 1,000 hectares, depending on numerous factors. Experiences show that the abundance of many wildlife species can be accurately determined in almost all forest ecosystems, offering further exciting analysis possibilities. However, research is needed to determine the error rate due to missed detections in various forest structures to calculate a corresponding correction factor.
Keywords: Wildlife management, Species monitoring
Full title: Determining Wildlife Abundance Using UAVs – Practical Experiences
Abstract: Accurate estimation of wildlife abundance is crucial for effective wildlife management and understanding their impact on vegetation and biodiversity. The use of Unmanned Aerial Vehicles (UAVs, drones) allows for precise monitoring of numerous wildlife species, though species and habitat-specific differences exist. Based on aerial surveys covering over 100,000 hectares using DJI M300 RTK and DJI M30T drones, practical experiences in UAV-based wildlife detection are presented, highlighting the influence of wildlife on vegetation patterns. Wildlife species react differently to UAVs; wild boars and roe deer rarely respond to drones flying at altitudes around and above 70 meters, whereas red deer and predators often detect UAVs and may exhibit flight behavior. Modern drones, given suitable conditions, can reliably detect and identify many wildlife species from over 100 meters away. The high-resolution optics of drones also provide additional information on gender and age distribution when needed. Drones enable GPS-accurate localization of animals, and combining this spatial distribution data with information on forest structure, hunting regimes, and vegetation patterns can yield many insightful correlations. Understanding these interactions helps in assessing the impact of wildlife on vegetation and overall biodiversity. The daily coverage area with the mentioned models ranges from approximately 300 to over 1,000 hectares, depending on numerous factors. Experiences show that the abundance of many wildlife species can be accurately determined in almost all forest ecosystems, offering further exciting analysis possibilities. However, research is needed to determine the error rate due to missed detections in various forest structures to calculate a corresponding correction factor.
Keywords: Wildlife management, Species monitoring
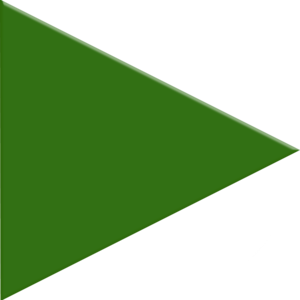
Presented by: Dr. Görres Grenzdörffer, Rostock University
Full title: Phenotyping of winter rye with drones
Abstract: In recent years, drones have become an increasingly important tool in plant breeding, particularly for phenotyping in the field, and many plant breeders would hardly want to be without them. After all, the drone offers many advantages. For example, a large number of plots can be flown over in just a few minutes and a wealth of information can be obtained without contact. The logistical effort for a single flight is low and the cost per flight is manageable. Provided the appropriate technical infrastructure is in place, the flight and evaluation of the drone data can be highly automated. The challenges of determining growth height with drones are crop-specific and also vary with phenological development. The literature mainly contains studies on wheat, rice, barley, maize, rapeseed and soybeans. Studies on winter rye are not to be found in the literature. Determining the crop height of rye is more challenging than for other cereals, as rye forms very long stems, which can also vary in height, and has a crop density that is significantly lower than that of wheat, for example. This means that the crop surface of winter rye is generally rougher than that of other cereals. This applies in particular to field trials with a large number of different genotypes. The study deals with the determination of the crop height of winter rye using drones and focuses on three aspects: 1. an investigation of various factors influencing the accuracy of photogrammetrically determined growth heights, 2. the comparative investigation of two camera systems (high-resolution RGB camera vs. low-resolution multispectral camera). A multi-temporal data set with 8 aerial surveys in the generative phase from the year 2022 is used for this purpose, and 3. an investigation of the importance of phenology on the accuracy of the CH measurements. For this purpose, a multi-temporal data set with 10 epochs from the 2023 vegetation period is used. The second focus of the work highlights the spatial change and dynamics of plant vitality obtained from the time series of multispectral drone data from 2022 and 2023. In detail: 1. correlation analyses are carried out to find out to what extent plant vitality changes spatially 2. when, or over which phenological period correltations of plant vitality and plant growth vs. yield exist The third focus of this article deals with various crop-related evaluations of the drone data with respect to winter rye breeding. The following investigations will be presented in more detail: 1. investigation of plant growth and plant vigor as a function of genotypes (experimental links), 2. investigation of the plant growth of semi-dwarfs, 3. differences in the growth and vigor of the plots are not only due to the genotypes, but are also a function of locally varying soil conditions within the trial fields.
Keywords: Phenotyping, Crop height, Crop vitality, Phenology
Full title: Phenotyping of winter rye with drones
Abstract: In recent years, drones have become an increasingly important tool in plant breeding, particularly for phenotyping in the field, and many plant breeders would hardly want to be without them. After all, the drone offers many advantages. For example, a large number of plots can be flown over in just a few minutes and a wealth of information can be obtained without contact. The logistical effort for a single flight is low and the cost per flight is manageable. Provided the appropriate technical infrastructure is in place, the flight and evaluation of the drone data can be highly automated. The challenges of determining growth height with drones are crop-specific and also vary with phenological development. The literature mainly contains studies on wheat, rice, barley, maize, rapeseed and soybeans. Studies on winter rye are not to be found in the literature. Determining the crop height of rye is more challenging than for other cereals, as rye forms very long stems, which can also vary in height, and has a crop density that is significantly lower than that of wheat, for example. This means that the crop surface of winter rye is generally rougher than that of other cereals. This applies in particular to field trials with a large number of different genotypes. The study deals with the determination of the crop height of winter rye using drones and focuses on three aspects: 1. an investigation of various factors influencing the accuracy of photogrammetrically determined growth heights, 2. the comparative investigation of two camera systems (high-resolution RGB camera vs. low-resolution multispectral camera). A multi-temporal data set with 8 aerial surveys in the generative phase from the year 2022 is used for this purpose, and 3. an investigation of the importance of phenology on the accuracy of the CH measurements. For this purpose, a multi-temporal data set with 10 epochs from the 2023 vegetation period is used. The second focus of the work highlights the spatial change and dynamics of plant vitality obtained from the time series of multispectral drone data from 2022 and 2023. In detail: 1. correlation analyses are carried out to find out to what extent plant vitality changes spatially 2. when, or over which phenological period correltations of plant vitality and plant growth vs. yield exist The third focus of this article deals with various crop-related evaluations of the drone data with respect to winter rye breeding. The following investigations will be presented in more detail: 1. investigation of plant growth and plant vigor as a function of genotypes (experimental links), 2. investigation of the plant growth of semi-dwarfs, 3. differences in the growth and vigor of the plots are not only due to the genotypes, but are also a function of locally varying soil conditions within the trial fields.
Keywords: Phenotyping, Crop height, Crop vitality, Phenology
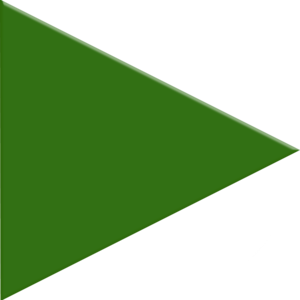
Presented by: Ms Xuerui Guo, Forschungszentrum Jülich GmbH
Full title: Using drones to enhance carbon fluxes determination in agricultural ecosystems
Abstract: Maintaining high yields while reducing carbon emissions is the focus of current agroecological research. The two carbon fluxes, gross primary productivity (GPP) and net ecosystem exchange (NEE), are two indicators for assessing the role of agricultural ecosystems in the carbon balance. Modelling and estimation of carbon fluxes in agricultural ecosystems have been established from different perspectives. Most process-based crop models simulate GPP and NEE by mechanically modelling carbon cycle processes, including soil organic matter decomposition, plant photosynthesis and respiration, dry matter partitioning, and so on. However, agricultural models are mostly one-dimensional and have limited spatial extension. The widely reported satellite GPP land products use plant-atmosphere interactions at the land surface to simulate canopy activities such as photosynthesis, but rarely consider below-ground respiration and soil carbon turnover processes, making it difficult to derive NEE from space observations alone. UAVs are a promising intermediate platform that can integrate high-resolution remote sensing observations and crop models and improve the spatial simulation of GPP and NEE in agricultural systems. In our study, we propose to integrate multispectral and thermal observations from UAVs with an agrocarbon model AgroC to improve the spatial and temporal simulation of GPP and NEE.
Keywords: Carbon flux, GPP, NEE, AgroC
Full title: Using drones to enhance carbon fluxes determination in agricultural ecosystems
Abstract: Maintaining high yields while reducing carbon emissions is the focus of current agroecological research. The two carbon fluxes, gross primary productivity (GPP) and net ecosystem exchange (NEE), are two indicators for assessing the role of agricultural ecosystems in the carbon balance. Modelling and estimation of carbon fluxes in agricultural ecosystems have been established from different perspectives. Most process-based crop models simulate GPP and NEE by mechanically modelling carbon cycle processes, including soil organic matter decomposition, plant photosynthesis and respiration, dry matter partitioning, and so on. However, agricultural models are mostly one-dimensional and have limited spatial extension. The widely reported satellite GPP land products use plant-atmosphere interactions at the land surface to simulate canopy activities such as photosynthesis, but rarely consider below-ground respiration and soil carbon turnover processes, making it difficult to derive NEE from space observations alone. UAVs are a promising intermediate platform that can integrate high-resolution remote sensing observations and crop models and improve the spatial simulation of GPP and NEE in agricultural systems. In our study, we propose to integrate multispectral and thermal observations from UAVs with an agrocarbon model AgroC to improve the spatial and temporal simulation of GPP and NEE.
Keywords: Carbon flux, GPP, NEE, AgroC
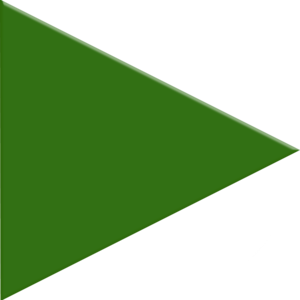
Presented by: Dr Juha Suomalainen, Finnish Geospatial Research Institute
Full title: High-accuracy empirical-line-method under natural illumination using advanced reflectance reference panel calibration
Abstract: In UAV-based optical remote sensing and field spectrometry, reflectance factor conversion is typically performed using one or more reflectance reference panels and the empirical line method. To be accurate, this method requires knowledge of the panel’s reflectance factor under current outdoor illumination conditions. This is generally not known as the panel manufacturers usually provide the reflectance factor only for laboratory calibration illumination (8°/H), which can differ significantly from the varying conditions experienced in natural outdoor illumination. To enable high-accuracy reflectance conversion of UAV data, we have developed a panel reflectance calibration procedure, libRadtran simulations, and a Python processing workflow to accurately predict the reflectance factor of a calibrated panel at any solar zenith angle under natural illumination conditions ("bluesky," "broken clouds," and "overcast"). In this poster, we (1) present the calibration methodology, (2) show the reflectance properties of selected panels, such as Spectralon 99% and Zenith Lite Polymers, and (3) demonstrate the magnitude and types of errors present in traditional UAV processes that our methodology can eliminate.
Keywords: Empirical line method, reflectance, BRDF, panel, libRadtran
Full title: High-accuracy empirical-line-method under natural illumination using advanced reflectance reference panel calibration
Abstract: In UAV-based optical remote sensing and field spectrometry, reflectance factor conversion is typically performed using one or more reflectance reference panels and the empirical line method. To be accurate, this method requires knowledge of the panel’s reflectance factor under current outdoor illumination conditions. This is generally not known as the panel manufacturers usually provide the reflectance factor only for laboratory calibration illumination (8°/H), which can differ significantly from the varying conditions experienced in natural outdoor illumination. To enable high-accuracy reflectance conversion of UAV data, we have developed a panel reflectance calibration procedure, libRadtran simulations, and a Python processing workflow to accurately predict the reflectance factor of a calibrated panel at any solar zenith angle under natural illumination conditions ("bluesky," "broken clouds," and "overcast"). In this poster, we (1) present the calibration methodology, (2) show the reflectance properties of selected panels, such as Spectralon 99% and Zenith Lite Polymers, and (3) demonstrate the magnitude and types of errors present in traditional UAV processes that our methodology can eliminate.
Keywords: Empirical line method, reflectance, BRDF, panel, libRadtran
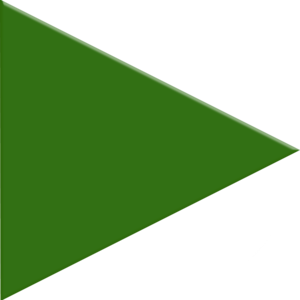
Presented by: Dr Agata Walicka, Aarhus University
Full title: Vegetation height estimation using UAV LiDAR
Abstract: Ensuring accurate, consistent, and fine-resolution measurements of vegetation (woody and herbaceous) height over large extents and across all habitat types has a pivotal role in monitoring natural ecosystems and mitigating the ongoing decline in biodiversity. However, manual measurement techniques are time-consuming, labor-intensive and rely on plot-based sampling. As a result, this approach does not provide a comprehensive understanding of vertical vegetation structure and condition across an entire area. These challenges can be overcome by employing laser scanning data as it generally enables penetration through vegetation layers. In this work we are developing methods enabling highly detailed and geographically comprehensive measurements of vegetation height using UAV laser scanning point cloud. The methods are developed and tested using almost 800 in-situ height measurements in a rewilding landscape of various vegetation types that include trees, shrubs and herbs. In this poster, we present results comparing methods that are based on direct point cloud analysis and various machine learning approaches.
Keywords: Biodiversity, Vegetation height, UAV LiDAR, Habitat condition metrics, machine learning
Full title: Vegetation height estimation using UAV LiDAR
Abstract: Ensuring accurate, consistent, and fine-resolution measurements of vegetation (woody and herbaceous) height over large extents and across all habitat types has a pivotal role in monitoring natural ecosystems and mitigating the ongoing decline in biodiversity. However, manual measurement techniques are time-consuming, labor-intensive and rely on plot-based sampling. As a result, this approach does not provide a comprehensive understanding of vertical vegetation structure and condition across an entire area. These challenges can be overcome by employing laser scanning data as it generally enables penetration through vegetation layers. In this work we are developing methods enabling highly detailed and geographically comprehensive measurements of vegetation height using UAV laser scanning point cloud. The methods are developed and tested using almost 800 in-situ height measurements in a rewilding landscape of various vegetation types that include trees, shrubs and herbs. In this poster, we present results comparing methods that are based on direct point cloud analysis and various machine learning approaches.
Keywords: Biodiversity, Vegetation height, UAV LiDAR, Habitat condition metrics, machine learning
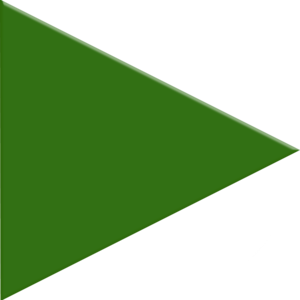
Presented by: Prof. Dr. Georg Bareth, University of Cologne (Geography)
Full title: UAV-derived Crop Height as an Estimator for Biomass, N-Concentration, and N-Uptake for Winter Wheat: Results of a 3-Years Data Set Analysis
Abstract: The Nitrogen Nutrition Index (NNI) is an established approach to determine N fertilizer demand on canopy and field scale. For accurate estimation of the NNI, reliable dry biomass (DB) and N concentration (Nconc) data are needed. Both usually are derived using optical remote or proximal sensing approaches. Another approach is to determine N-uptake (Nup). For the latter, again reliable data on dry DB and Nconc is demanded. In this contribution, we investigate a paradigm change in providing non-destructive DB, Nconc, and Nup estimates by using non-optical data analyses but structural information extraction. The three key hypotheses are that (i) crop height from UAV images using a Structure from Motion and Multiview Stereopsis (SfM/MVS) workflow serves as a very robust estimator for DB, (ii) Nconc is negatively correlated over the growing season to DB, and (iii) DB therefore is the dominating parameter in determining Nup. This would result in the main research question if UAV-derived crop height (ÚAV-CH), as a robust estimator for DB, can be used to directly estimate Nconc and Nup, hence spatial UAV-CH is a mixed signal of crop height and density. The results from a 3-years effort in UAV- and in-situ data acquisition and analyses support the proposed paradigm change. For each of the three years 2020, 2021, and 2022, DB can be estimated using UAV-CH, having a R2 of 0.94, 0.91, and 0.92, respectively. For Nup the results are not as promising on a yearly analysis having R2 of 0.72, 0.58, and 0.48, respectively, but are comparable to optical approaches. However, for fertilizer recommendation, the performance on daily level is of more importance. Surprisingly, the proposed approach seems to overcome this limitation of optical sensing analysis showing R2 of daily analysis (approx. every 14 days over 3 growing periods) for N uptake between 0.57 and 0.82, and for Nconc between 0.24 and 0.78. We conclude that UAV-CH can be used as a strong estimator for DB and as a moderate but robust estimator for Nup.
Keywords: UAV, nitrogen uptake, biomass, winter wheat, plant height
Full title: UAV-derived Crop Height as an Estimator for Biomass, N-Concentration, and N-Uptake for Winter Wheat: Results of a 3-Years Data Set Analysis
Abstract: The Nitrogen Nutrition Index (NNI) is an established approach to determine N fertilizer demand on canopy and field scale. For accurate estimation of the NNI, reliable dry biomass (DB) and N concentration (Nconc) data are needed. Both usually are derived using optical remote or proximal sensing approaches. Another approach is to determine N-uptake (Nup). For the latter, again reliable data on dry DB and Nconc is demanded. In this contribution, we investigate a paradigm change in providing non-destructive DB, Nconc, and Nup estimates by using non-optical data analyses but structural information extraction. The three key hypotheses are that (i) crop height from UAV images using a Structure from Motion and Multiview Stereopsis (SfM/MVS) workflow serves as a very robust estimator for DB, (ii) Nconc is negatively correlated over the growing season to DB, and (iii) DB therefore is the dominating parameter in determining Nup. This would result in the main research question if UAV-derived crop height (ÚAV-CH), as a robust estimator for DB, can be used to directly estimate Nconc and Nup, hence spatial UAV-CH is a mixed signal of crop height and density. The results from a 3-years effort in UAV- and in-situ data acquisition and analyses support the proposed paradigm change. For each of the three years 2020, 2021, and 2022, DB can be estimated using UAV-CH, having a R2 of 0.94, 0.91, and 0.92, respectively. For Nup the results are not as promising on a yearly analysis having R2 of 0.72, 0.58, and 0.48, respectively, but are comparable to optical approaches. However, for fertilizer recommendation, the performance on daily level is of more importance. Surprisingly, the proposed approach seems to overcome this limitation of optical sensing analysis showing R2 of daily analysis (approx. every 14 days over 3 growing periods) for N uptake between 0.57 and 0.82, and for Nconc between 0.24 and 0.78. We conclude that UAV-CH can be used as a strong estimator for DB and as a moderate but robust estimator for Nup.
Keywords: UAV, nitrogen uptake, biomass, winter wheat, plant height
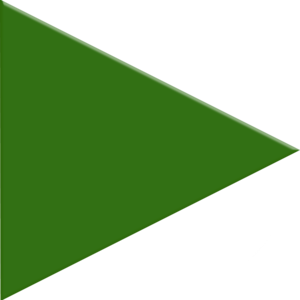
Presented by: Dr Dominic Fawcett, Swiss Federal Institute for Forest Snow and Landscape Research WSL
Full title: The potential of imaging spectroscopy data for resolving differences in tree vitality across scales
Abstract: European forests are facing increasing pressure from environmental stresses which are exacerbated by climate change. As part of the Forestward Observatory to Secure Resilience of European Forests (FORWARDS) we seek to bridge the gap between ground-based measurements of tree vitality and remote sensing methods. Multi- and hyperspectral reflectance data have the potential to map pre-visual vulnerability symptoms due to changes in leaf water and pigment contents. The interpretation of spectral indicators can, however, vary at leaf, crown and stand level, as well as between species and forest structure, thus requiring a multi-scale approach to investigate the suitability of hyperspectral measurements to differentiate stressed from less stressed trees and forest stands. We make use of multiple scales of remote sensing data to investigate 1) which spectral indicators are linked to different measurements of spatially varying tree vitality and 2) how such indicators behave at crown, stand and landscape level, informing on approaches that could be promising for operational use in Europe-wide forest vitality monitoring. We present first results from a pilot phase of this project focused on three intensively monitored sites in Switzerland, including a rainfall exclusion experiment for investigating drought stress. For these sites, leaf and tree-level data in the field as well as acquisitions by drone (<0.1 m) and airborne (1 m) hyperspectral sensors were conducted in August 2023, and airborne data were used to simulate hyperspectral satellite acquisitions (30 m). Preliminary results show that while there appear to be significant links between some spectral indices and indicators of drought stress at tree level, challenges remain when aggregating over structurally and species diverse forest stands.
Keywords: Hyperspectral, UAV, Forest, Vitality
Full title: The potential of imaging spectroscopy data for resolving differences in tree vitality across scales
Abstract: European forests are facing increasing pressure from environmental stresses which are exacerbated by climate change. As part of the Forestward Observatory to Secure Resilience of European Forests (FORWARDS) we seek to bridge the gap between ground-based measurements of tree vitality and remote sensing methods. Multi- and hyperspectral reflectance data have the potential to map pre-visual vulnerability symptoms due to changes in leaf water and pigment contents. The interpretation of spectral indicators can, however, vary at leaf, crown and stand level, as well as between species and forest structure, thus requiring a multi-scale approach to investigate the suitability of hyperspectral measurements to differentiate stressed from less stressed trees and forest stands. We make use of multiple scales of remote sensing data to investigate 1) which spectral indicators are linked to different measurements of spatially varying tree vitality and 2) how such indicators behave at crown, stand and landscape level, informing on approaches that could be promising for operational use in Europe-wide forest vitality monitoring. We present first results from a pilot phase of this project focused on three intensively monitored sites in Switzerland, including a rainfall exclusion experiment for investigating drought stress. For these sites, leaf and tree-level data in the field as well as acquisitions by drone (<0.1 m) and airborne (1 m) hyperspectral sensors were conducted in August 2023, and airborne data were used to simulate hyperspectral satellite acquisitions (30 m). Preliminary results show that while there appear to be significant links between some spectral indices and indicators of drought stress at tree level, challenges remain when aggregating over structurally and species diverse forest stands.
Keywords: Hyperspectral, UAV, Forest, Vitality
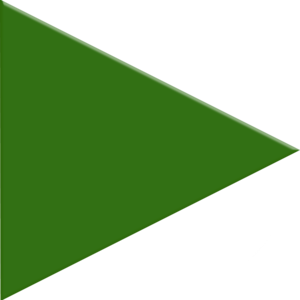
Presented by: Dr Na Wang, University of Melbourne
Full title: An approach for compensating atmospheric effects within the O2-A band for the retrieval of solar-induced fluorescence from high-altitude UAV platforms
Abstract: Solar induced fluorescence (SIF) has been used as a promising proxy of photosynthetic activity at field to global scale. Various SIF retrieval strategies have been developed for flux tower, UAV, and piloted aircraft applications, aiming to extract SIF signals from upwelling at-sensor radiance by leveraging oxygen absorption spectral regions. Despite their high sensitivity to detect SIF, these regions are largely influenced by atmospheric effects, necessitating careful consideration to ensure reliable SIF retrieval methods. Currently, atmospheric correction algorithms are applied to the sensor-level upwelling radiance. This process involves estimating several atmospheric parameters, such as path radiance, transmittance, and spherical albedo, using an atmospheric radiative transfer model. Nevertheless, accurately estimating all the required atmospheric parameters poses a challenge and can lead to substantial inaccuracies in SIF estimates if these parameters are not precisely determined. In this work, we propose a novel and direct method to mitigate atmospheric effects in SIF quantification without the need for atmospheric correction of the at-sensor radiance. Our method involves using only two atmospheric parameters: upward direct transmittance in the sensor observing direction and upward diffused transmittance from top of canopy (TOC) to sensor height to simulate upwelling irradiance at the sensor level. Upwelling irradiance refers to the irradiance that reaches the canopy but does not interact with it, instead traveling directly upward to the sensor. We hypothesize that upwelling irradiance, and upwelling radiance, including SIF, undergo similar atmospheric influences from the canopy to the sensor level. Therefore, significant mitigation of atmospheric effects on SIF retrieval from upwelling irradiance and radiance can be achieved. To test this hypothesis, we utilized the radiative transfer models SCOPE and MODTRAN6 by considering the heterogeneity of the landscape, non-Lambertian reflectance of the land surface, and atmospheric adjacency effects. Upwelling irradiance at the sensor level was simulated to quantify SIF from sensor-level upward radiance. Three scenarios under clear sky conditions were defined for the analysis. In Scenario 1, TOC SIF was quantified using TOC downwelling irradiance and TOC upwelling radiance, serving as the reference SIF. Scenario 2, involved quantifying SIF using downwelling TOC irradiance and upwelling sensor radiance at altitudes of 350 m, 800 m, and 1200 m. In Scenario 3, SIF was derived from upward sensor irradiance and radiance at the same altitudes. Our findings indicate that SIF from Scenario 2 showed good correlation with TOC SIF from Scenario 1 at 350 m (R² = 0.84), but correlations weakened at higher altitudes (R² < 0.4 at 800 m and 1200 m). Conversely, SIF from Scenario 3 exhibited robust correlations with TOC SIF from Scenario 1, with R² values of 0.95, 0.94, and 0.93 at 350 m, 800 m, and 1200 m, respectively. These results underscore the potential of using upwelling irradiance for SIF quantification from sensor-level radiance. This approach shows potential for various SIF measurement platforms, including UAV-based and airborne systems, where simulated or ground-based downwelling TOC irradiance measurements are commonly used for SIF quantification.
Keyword: Solar-induced fluorescence; Atmospheric correction; Upwelling irradiance; Radiative transfer models
Full title: An approach for compensating atmospheric effects within the O2-A band for the retrieval of solar-induced fluorescence from high-altitude UAV platforms
Abstract: Solar induced fluorescence (SIF) has been used as a promising proxy of photosynthetic activity at field to global scale. Various SIF retrieval strategies have been developed for flux tower, UAV, and piloted aircraft applications, aiming to extract SIF signals from upwelling at-sensor radiance by leveraging oxygen absorption spectral regions. Despite their high sensitivity to detect SIF, these regions are largely influenced by atmospheric effects, necessitating careful consideration to ensure reliable SIF retrieval methods. Currently, atmospheric correction algorithms are applied to the sensor-level upwelling radiance. This process involves estimating several atmospheric parameters, such as path radiance, transmittance, and spherical albedo, using an atmospheric radiative transfer model. Nevertheless, accurately estimating all the required atmospheric parameters poses a challenge and can lead to substantial inaccuracies in SIF estimates if these parameters are not precisely determined. In this work, we propose a novel and direct method to mitigate atmospheric effects in SIF quantification without the need for atmospheric correction of the at-sensor radiance. Our method involves using only two atmospheric parameters: upward direct transmittance in the sensor observing direction and upward diffused transmittance from top of canopy (TOC) to sensor height to simulate upwelling irradiance at the sensor level. Upwelling irradiance refers to the irradiance that reaches the canopy but does not interact with it, instead traveling directly upward to the sensor. We hypothesize that upwelling irradiance, and upwelling radiance, including SIF, undergo similar atmospheric influences from the canopy to the sensor level. Therefore, significant mitigation of atmospheric effects on SIF retrieval from upwelling irradiance and radiance can be achieved. To test this hypothesis, we utilized the radiative transfer models SCOPE and MODTRAN6 by considering the heterogeneity of the landscape, non-Lambertian reflectance of the land surface, and atmospheric adjacency effects. Upwelling irradiance at the sensor level was simulated to quantify SIF from sensor-level upward radiance. Three scenarios under clear sky conditions were defined for the analysis. In Scenario 1, TOC SIF was quantified using TOC downwelling irradiance and TOC upwelling radiance, serving as the reference SIF. Scenario 2, involved quantifying SIF using downwelling TOC irradiance and upwelling sensor radiance at altitudes of 350 m, 800 m, and 1200 m. In Scenario 3, SIF was derived from upward sensor irradiance and radiance at the same altitudes. Our findings indicate that SIF from Scenario 2 showed good correlation with TOC SIF from Scenario 1 at 350 m (R² = 0.84), but correlations weakened at higher altitudes (R² < 0.4 at 800 m and 1200 m). Conversely, SIF from Scenario 3 exhibited robust correlations with TOC SIF from Scenario 1, with R² values of 0.95, 0.94, and 0.93 at 350 m, 800 m, and 1200 m, respectively. These results underscore the potential of using upwelling irradiance for SIF quantification from sensor-level radiance. This approach shows potential for various SIF measurement platforms, including UAV-based and airborne systems, where simulated or ground-based downwelling TOC irradiance measurements are commonly used for SIF quantification.
Keyword: Solar-induced fluorescence; Atmospheric correction; Upwelling irradiance; Radiative transfer models
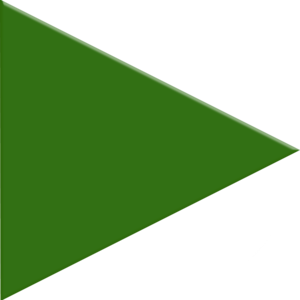
Presented by: MSc Sofia Choza Farias, Forschungszentrum Juelich
Full title: Drone-based solar induced chlorophyll fluorescence (SIF) for assessing photosynthetic response in different crop varieties
Abstract: Solar Induced Fluorescence (SIF) signal retrieved from plants provides a promising approach to monitor vegetation health and status. Hence, remote sensing of SIF has rapidly advanced into new techniques and methods to gain valuable information of photosynthetic activity in plants. Current remote sensing techniques enable the measurement of SIF across various biological, spatial and temporal scales. This optical signal can be remotely analyzed using advanced spectral sensors and algorithms that differentiate it from reflected ambient light. The non-invasive detection of red to far-red SIF allows for repeated sampling to obtain detailed spatio-temporal information about photosynthetic light responses and the steady-state behavior of vegetation. Advances in sensor technology, retrieval methods and modelling are driving rapid progress in this field. This study aims to integrate two distinct optical SIF-measuring systems: a novel camera system (SIFcam) with two specific spectral bands (757 and 760nm) and a non-imaging hyperspectral sensor that provides high spectral resolution data (AirFloX). The goal is to determine which approach, or a combination of both, is most suitable for systematically detecting the spatio-temporal dynamics of SIF in agricultural crops in a field experiment with various crop trials, located in Campus Klein Altendorf, an experimental site of the University of Bonn. This opens the possibility of applying this new methodology to use SIF as a non-invasive indicator for photosynthetic efficiency and vegetation stress on bigger fields and to expand our approach to conventional farming practices.
Keywords: Solar Induced Fluorescence (SIF), Photosynthesis, UAV, Agriculture, Remote Sensing
Full title: Drone-based solar induced chlorophyll fluorescence (SIF) for assessing photosynthetic response in different crop varieties
Abstract: Solar Induced Fluorescence (SIF) signal retrieved from plants provides a promising approach to monitor vegetation health and status. Hence, remote sensing of SIF has rapidly advanced into new techniques and methods to gain valuable information of photosynthetic activity in plants. Current remote sensing techniques enable the measurement of SIF across various biological, spatial and temporal scales. This optical signal can be remotely analyzed using advanced spectral sensors and algorithms that differentiate it from reflected ambient light. The non-invasive detection of red to far-red SIF allows for repeated sampling to obtain detailed spatio-temporal information about photosynthetic light responses and the steady-state behavior of vegetation. Advances in sensor technology, retrieval methods and modelling are driving rapid progress in this field. This study aims to integrate two distinct optical SIF-measuring systems: a novel camera system (SIFcam) with two specific spectral bands (757 and 760nm) and a non-imaging hyperspectral sensor that provides high spectral resolution data (AirFloX). The goal is to determine which approach, or a combination of both, is most suitable for systematically detecting the spatio-temporal dynamics of SIF in agricultural crops in a field experiment with various crop trials, located in Campus Klein Altendorf, an experimental site of the University of Bonn. This opens the possibility of applying this new methodology to use SIF as a non-invasive indicator for photosynthetic efficiency and vegetation stress on bigger fields and to expand our approach to conventional farming practices.
Keywords: Solar Induced Fluorescence (SIF), Photosynthesis, UAV, Agriculture, Remote Sensing
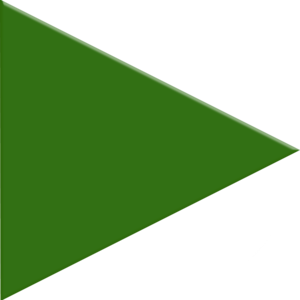
Presented by: Ms Jorita Krieger, Julius Kühn-Institut
Full title: UAV-assisted detection of heterogeneity in agricultural field trials
Abstract: The heterogeneity observed within agricultural field trials can be attributed to a multitude of factors, including the presence of small-scale heterogeneous soil conditions and the influence of biotic and abiotic stressors. The resulting differences within the plot can be rapidly and objectively documented using unmanned aerial vehicle (UAV) sensor technology. The objective of this study is to document and evaluate the heterogeneity present within plots. Therefore, several flights with the multispectral sensor MicaSense Altum PT using a DJI M350 RTK were conducted during the vegetation period in 2024 across different crops, including maize, sorghum, potatoes and winter cereals. Furthermore, heterogeneity indices will be developed based on the height of the vegetation and its reflectivity. These indices will enhance our understanding of differences in vegetation development and yield formation.
Keywords: Heterogeneity, Vegetation indices, Multispectral, Crop height
Full title: UAV-assisted detection of heterogeneity in agricultural field trials
Abstract: The heterogeneity observed within agricultural field trials can be attributed to a multitude of factors, including the presence of small-scale heterogeneous soil conditions and the influence of biotic and abiotic stressors. The resulting differences within the plot can be rapidly and objectively documented using unmanned aerial vehicle (UAV) sensor technology. The objective of this study is to document and evaluate the heterogeneity present within plots. Therefore, several flights with the multispectral sensor MicaSense Altum PT using a DJI M350 RTK were conducted during the vegetation period in 2024 across different crops, including maize, sorghum, potatoes and winter cereals. Furthermore, heterogeneity indices will be developed based on the height of the vegetation and its reflectivity. These indices will enhance our understanding of differences in vegetation development and yield formation.
Keywords: Heterogeneity, Vegetation indices, Multispectral, Crop height
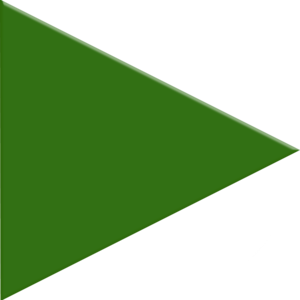
Presented by: Dr.-Ing. Anna Göritz, University of Freiburg
Full title: Assessing forest ecosystems with UAV based remote sensing – from laser scanning towards an active Chlorophyll fluorescence LiDAR.
Abstract: Forests play an important role in the global carbon cycle and offer a broad range of socio-economic ecosystem services. For a better understanding of the effects of different stressors on these structurally complex and spatially heterogeneous ecosystems, we aim to develop a novel instrumentation for tracking forest vitality using active UAV based sensing of Chlorophyll fluorescence. This work is embedded in a collaborative research centre called “Ecosense”, which aims at the development of a smart, autonomous sensor network for the quantification and modelling of spatio-temporal dynamics of different ecosystem processes such as water or carbon fluxes in forest environments. In this presentation, we introduce to our interdisciplinary research project, give insights into the sensor development process and present first results from our studies with UAV based laser scanning using commercial equipment over a natural temperate forest
Keywords: UAV, Forest, Chlorophyll fluorescence, LiDAR, Vitality
Full title: Assessing forest ecosystems with UAV based remote sensing – from laser scanning towards an active Chlorophyll fluorescence LiDAR.
Abstract: Forests play an important role in the global carbon cycle and offer a broad range of socio-economic ecosystem services. For a better understanding of the effects of different stressors on these structurally complex and spatially heterogeneous ecosystems, we aim to develop a novel instrumentation for tracking forest vitality using active UAV based sensing of Chlorophyll fluorescence. This work is embedded in a collaborative research centre called “Ecosense”, which aims at the development of a smart, autonomous sensor network for the quantification and modelling of spatio-temporal dynamics of different ecosystem processes such as water or carbon fluxes in forest environments. In this presentation, we introduce to our interdisciplinary research project, give insights into the sensor development process and present first results from our studies with UAV based laser scanning using commercial equipment over a natural temperate forest
Keywords: UAV, Forest, Chlorophyll fluorescence, LiDAR, Vitality
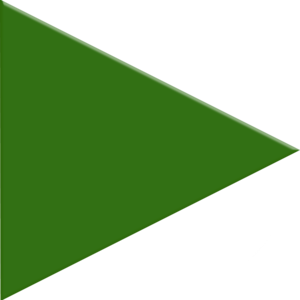
Presented by: Miss Jana Wieme, Ghent University - ILVO
Full title: Supevised detection of Alternaria solani on ultra-high-resolution UAV images of potato fields
Abstract: Alternaria solani, a destructive foliar pathogen causing premature defoliation of potato plants, is currently managed by applying detrimental crop protection products, guided by weather predictions and visual observations. Automated detection could enhance the accuracy of mapping Alternaria solani, thereby optimizing the use of crop protection products. Recent advances in precision agriculture highlight deep learning, particularly Convolutional Neural Networks (CNNs), though they face challenges like in-field robustness due to limited or controlled-environment training data. We gathered ultra-high-resolution modified RGB UAV imagery of potato crops, capturing both symptomatic and non-symptomatic plants across different years and disease stages. Our CNN-based model for in-field Alternaria detection, posed as a binary classification task, achieves similar accuracy to state-of-the-art models but with lower inference time, enhancing practical use. Using training data from 2019-2021 and test data from 2022, our model achieves an F1 score of 0.93. We also assess the impact of dataset size and class imbalance on accuracy.
Keywords: Alternaria solani, Supervised deep learning, Potato fields, UAV sub-mm resolution
Full title: Supevised detection of Alternaria solani on ultra-high-resolution UAV images of potato fields
Abstract: Alternaria solani, a destructive foliar pathogen causing premature defoliation of potato plants, is currently managed by applying detrimental crop protection products, guided by weather predictions and visual observations. Automated detection could enhance the accuracy of mapping Alternaria solani, thereby optimizing the use of crop protection products. Recent advances in precision agriculture highlight deep learning, particularly Convolutional Neural Networks (CNNs), though they face challenges like in-field robustness due to limited or controlled-environment training data. We gathered ultra-high-resolution modified RGB UAV imagery of potato crops, capturing both symptomatic and non-symptomatic plants across different years and disease stages. Our CNN-based model for in-field Alternaria detection, posed as a binary classification task, achieves similar accuracy to state-of-the-art models but with lower inference time, enhancing practical use. Using training data from 2019-2021 and test data from 2022, our model achieves an F1 score of 0.93. We also assess the impact of dataset size and class imbalance on accuracy.
Keywords: Alternaria solani, Supervised deep learning, Potato fields, UAV sub-mm resolution
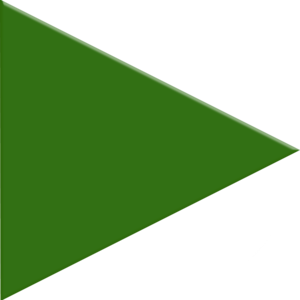
Presented by: PhD Aya Amar, Ghent University
Full title: Integrating UAV and Satellite Imagery with Field Measurements to Estimate Potato Crop Losses Using a Crop Growth Model
Abstract: Accurate estimation of crop losses is crucial for enhancing agricultural management and decision-making processes. This study integrates satellite imagery, UAV (Unmanned Aerial Vehicle) data, and field measurements to estimate potato losses at the production stage. Utilizing high-resolution multispectral and thermal sensors mounted on UAVs, along with Sentinel-2 satellite data, we monitored potato crop growth throughout the growing season. Ground truth data, including SPAD chlorophyll readings and detailed assessments of tuber yield and quality, were collected to validate the remote sensing data. These datasets were input into a robust crop growth model to simulate both optimal and stress conditions. By comparing the attainable yield under ideal conditions to the actual yield observed, we quantified the yield gap attributable to various stress factors. This multidisciplinary approach provides a comprehensive framework for assessing crop health and productivity, enabling more informed agricultural practices and loss mitigation strategies.
Keywords: Crop growth model, Production loss, UAV, Multispectral sensors, Thermal sensor
Full title: Integrating UAV and Satellite Imagery with Field Measurements to Estimate Potato Crop Losses Using a Crop Growth Model
Abstract: Accurate estimation of crop losses is crucial for enhancing agricultural management and decision-making processes. This study integrates satellite imagery, UAV (Unmanned Aerial Vehicle) data, and field measurements to estimate potato losses at the production stage. Utilizing high-resolution multispectral and thermal sensors mounted on UAVs, along with Sentinel-2 satellite data, we monitored potato crop growth throughout the growing season. Ground truth data, including SPAD chlorophyll readings and detailed assessments of tuber yield and quality, were collected to validate the remote sensing data. These datasets were input into a robust crop growth model to simulate both optimal and stress conditions. By comparing the attainable yield under ideal conditions to the actual yield observed, we quantified the yield gap attributable to various stress factors. This multidisciplinary approach provides a comprehensive framework for assessing crop health and productivity, enabling more informed agricultural practices and loss mitigation strategies.
Keywords: Crop growth model, Production loss, UAV, Multispectral sensors, Thermal sensor
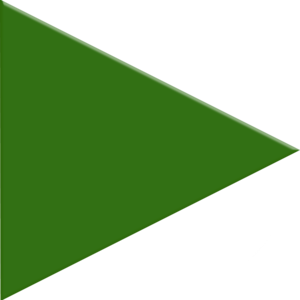
Presented by: Kilian Trimborn, Ansgar Dreier, Lasse Klingbeil, Heiner Kuhlmann
Full title: Leaf area index determination using UAV-based laser scanning
Abstract: The leaf area index (LAI: leaf area per ground area) is a very important phenotypic trait, used in precision agriculture. Conventional Methods for estimating the LAI are often time consuming and only valid for small spatial scales. Accurate determination of LAI of large fields with high temporal and spatial resolution remains a challenge. In our poster we present how to efficiently estimate the LAI using UAV-based laser scanning. UAVs are able to perform laser scans of large fields in just a few minutes. The LAI can be automatically calculated from the raw point cloud data with a user defined spatial resolution. A key property of laser scanning is the capability of penetrating the canopy. In areas with lower LAI values the laser rays can reach deeper into the canopy than in denser areas. A ray tracing algorithm in combination with Beers Law is performed to analyze the attenuation of the beams in the crop and to estimate the LAI. The distribution of the leaf angles also influences the penetration of the rays. We show how the LAI estimation can be optimized integrating the true leaf angle distribution. Our approach is first evaluated, simulating laser scans on virtual fields with known LAI and leaf angle distribution. Moreover, we estimated LAI from real data over a whole growing season for different crops with a spatial resolution of 1x1m.
Keywords: UAV-based laser scanning, leaf area index (LAI)
Full title: Leaf area index determination using UAV-based laser scanning
Abstract: The leaf area index (LAI: leaf area per ground area) is a very important phenotypic trait, used in precision agriculture. Conventional Methods for estimating the LAI are often time consuming and only valid for small spatial scales. Accurate determination of LAI of large fields with high temporal and spatial resolution remains a challenge. In our poster we present how to efficiently estimate the LAI using UAV-based laser scanning. UAVs are able to perform laser scans of large fields in just a few minutes. The LAI can be automatically calculated from the raw point cloud data with a user defined spatial resolution. A key property of laser scanning is the capability of penetrating the canopy. In areas with lower LAI values the laser rays can reach deeper into the canopy than in denser areas. A ray tracing algorithm in combination with Beers Law is performed to analyze the attenuation of the beams in the crop and to estimate the LAI. The distribution of the leaf angles also influences the penetration of the rays. We show how the LAI estimation can be optimized integrating the true leaf angle distribution. Our approach is first evaluated, simulating laser scans on virtual fields with known LAI and leaf angle distribution. Moreover, we estimated LAI from real data over a whole growing season for different crops with a spatial resolution of 1x1m.
Keywords: UAV-based laser scanning, leaf area index (LAI)
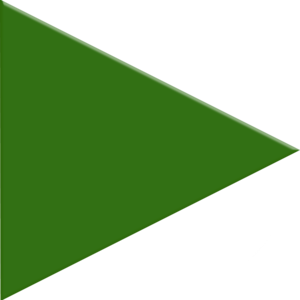
Presented by: Markus Adam, Max Marquardt, Marlin M. Mueller, Steffen Dietenberger, Julian Leiber, Clémence Dubois, Christian Thiel
Full title: Enhancing Coastal Resilience: UAV-based Deep Learning for Advanced Dike and Vegetation Monitoring
Abstract: This study presents an innovative approach to coastal protection and ecosystem management using Unmanned Aerial Vehicle (UAV) technology coupled with advanced deep learning algorithms. Focusing on the North Sea coast of Germany, our research aims to develop a high-resolution monitoring system for dikes and their associated vegetation, addressing the pressing challenges posed by climate change and sea-level rise. Utilizing a DJI Mavic 3 Enterprise drone, we captured detailed imagery of dike areas and foreland structures in two distinct study sites: a dike section in Dorum-Neufeld and the ecologically significant floating moor of Sehestedt. These areas represent critical interfaces between land and sea, where effective monitoring is essential for both coastal defense and habitat preservation. The data acquisition process involved 3-hour flight missions at an altitude of 80 and 120 meters, covering areas once in October 2023 and once in April 2024. Our methodology employs a U-Net algorithm, to classify and analyze various landscape features, including vegetation cover, maintenance paths, water bodies, and soil conditions. The classification workflow consisted of three main stages: (1) exporting training data using 256x256 pixel patches with 50% overlap, (2) training the deep learning model using ResNet-34 as the backbone, and (3) applying the trained model for pixel-wise classification. We explored various input combinations, including RGB imagery alone and in conjunction with elevation and slope data, to enhance classification accuracy. This approach allows for fast and precise delineation of dike structures and vegetation patterns, offering insights into the health and stability of these coastal protection systems. By providing detailed, up-to-date information on dike integrity and vegetation dynamics, our method enables more targeted and efficient maintenance practices. This not only enhances the resilience of coastal communities against flooding and erosion but also supports the preservation of unique coastal ecosystems. This research contributes to the growing field of environmental monitoring by showcasing the potential of UAV-based remote sensing and artificial intelligence in addressing complex ecological and infrastructural challenges. As coastal regions worldwide face increasing threats from climate change, our methodology offers a powerful tool for informed decision-making and adaptive management strategies.
Full title: Enhancing Coastal Resilience: UAV-based Deep Learning for Advanced Dike and Vegetation Monitoring
Abstract: This study presents an innovative approach to coastal protection and ecosystem management using Unmanned Aerial Vehicle (UAV) technology coupled with advanced deep learning algorithms. Focusing on the North Sea coast of Germany, our research aims to develop a high-resolution monitoring system for dikes and their associated vegetation, addressing the pressing challenges posed by climate change and sea-level rise. Utilizing a DJI Mavic 3 Enterprise drone, we captured detailed imagery of dike areas and foreland structures in two distinct study sites: a dike section in Dorum-Neufeld and the ecologically significant floating moor of Sehestedt. These areas represent critical interfaces between land and sea, where effective monitoring is essential for both coastal defense and habitat preservation. The data acquisition process involved 3-hour flight missions at an altitude of 80 and 120 meters, covering areas once in October 2023 and once in April 2024. Our methodology employs a U-Net algorithm, to classify and analyze various landscape features, including vegetation cover, maintenance paths, water bodies, and soil conditions. The classification workflow consisted of three main stages: (1) exporting training data using 256x256 pixel patches with 50% overlap, (2) training the deep learning model using ResNet-34 as the backbone, and (3) applying the trained model for pixel-wise classification. We explored various input combinations, including RGB imagery alone and in conjunction with elevation and slope data, to enhance classification accuracy. This approach allows for fast and precise delineation of dike structures and vegetation patterns, offering insights into the health and stability of these coastal protection systems. By providing detailed, up-to-date information on dike integrity and vegetation dynamics, our method enables more targeted and efficient maintenance practices. This not only enhances the resilience of coastal communities against flooding and erosion but also supports the preservation of unique coastal ecosystems. This research contributes to the growing field of environmental monitoring by showcasing the potential of UAV-based remote sensing and artificial intelligence in addressing complex ecological and infrastructural challenges. As coastal regions worldwide face increasing threats from climate change, our methodology offers a powerful tool for informed decision-making and adaptive management strategies.
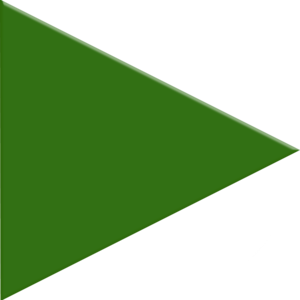
Presented by: Steffen Dietenberger, Marlin M. Mueller, Markus Adam, Boris Stöcker, Felix Bachmann, Clémence Dubois, Christian Thiel
Full title: Digital Forest Inventory based on UAV Imagery
Abstract: Accurate and up-to-date data are essential for sustainable forest management and effective monitoring. The German forestry sector faces several challenges, including the impacts of climate change, staff shortages, a trend towards more diverse forest stands, and increasing digitalization. To address these challenges, this study analyses the use of UAV-based Structure from Motion (SfM) techniques for digital forest inventories. UAV-SfM data products, such as 3D point clouds and orthomosaics, were generated from a study site in Hainich National Park, Germany. These data were used to create a comprehensive digital representation of the forest structure, encompassing canopy, stems, and ground components. Various flight configurations, including nadir and oblique camera angles, and different acquisition times (leaf-on and leaf-off conditions) were combined to enhance data quality. The resulting SfM data products were used for the automated derivation of forest parameters such as tree stem positions, individual tree crown delineation (ITCD), diameter at breast height (DBH), and coarse woody debris (CWD). In leaf-off conditions, a clustering algorithm was applied to segment the point cloud and extract tree stem positions with high precision of 86.7%. Lower recall values (69.7%) indicate that existing trees were missed. These positions served as markers for crown delineation in leaf-on data, slightly improving delineation accuracy. DBH was derived using classified cast shadows, resulting in an overall accuracy of 98.6% for shadow classification and an RMSE of 17 cm for DBH values. For deadwood detection, leaf-off orthomosaics of the forest floor were used to classify CWD objects.
Full title: Digital Forest Inventory based on UAV Imagery
Abstract: Accurate and up-to-date data are essential for sustainable forest management and effective monitoring. The German forestry sector faces several challenges, including the impacts of climate change, staff shortages, a trend towards more diverse forest stands, and increasing digitalization. To address these challenges, this study analyses the use of UAV-based Structure from Motion (SfM) techniques for digital forest inventories. UAV-SfM data products, such as 3D point clouds and orthomosaics, were generated from a study site in Hainich National Park, Germany. These data were used to create a comprehensive digital representation of the forest structure, encompassing canopy, stems, and ground components. Various flight configurations, including nadir and oblique camera angles, and different acquisition times (leaf-on and leaf-off conditions) were combined to enhance data quality. The resulting SfM data products were used for the automated derivation of forest parameters such as tree stem positions, individual tree crown delineation (ITCD), diameter at breast height (DBH), and coarse woody debris (CWD). In leaf-off conditions, a clustering algorithm was applied to segment the point cloud and extract tree stem positions with high precision of 86.7%. Lower recall values (69.7%) indicate that existing trees were missed. These positions served as markers for crown delineation in leaf-on data, slightly improving delineation accuracy. DBH was derived using classified cast shadows, resulting in an overall accuracy of 98.6% for shadow classification and an RMSE of 17 cm for DBH values. For deadwood detection, leaf-off orthomosaics of the forest floor were used to classify CWD objects.
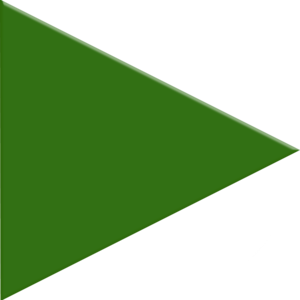
Presented by: Lucas Casuccio, Prof. Dr. Zbyněk Malenovský , Prof. Dr. Ribana Roscher
Full title: Combining in-silico multispectral imagery from 3D radiative transfer with machine learning for retrieval of plant biochemical traits.
Abstract: This study presents development of a novel drone-based approach for high-throughput plant phenotyping. This approach uses labelled multispectral imagery generated by the three-dimensional (3D) discrete anisotropic radiative transfer (DART) model, to train a machine learning algorithm retrieving biochemical traits of individual plant leaves from complementary high spatial resolution optical imagery acquired with an uncrewed aircraft system (UAS). Traditional field phenotyping methods are often limited by the need for extensive and invasive sampling practices, costly equipment, and specific technical requirements to capture the complexity of plant responses under controlled or varying environmental conditions. A number of remote sensing phenotyping methods rely on empirical relationships between biochemical traits and vegetation optical indices, calibrated and validated with field measurements. Although some studies have used radiative transfer modelling for phenotyping purposes, they frequently do not account for full 3D structural heterogeneity of complex canopies. Our method addresses the spatial variability in crop traits and the often non-representative nature of field measurements. It is expected to enhance the accuracy and efficiency of phenotyping practices by using the full 3D DART to simulate multispectral data with 1 cm spatial sampling distance, mimicking the UAS images of an off-the-shelf 10-band multispectral sensor from the Micasense company. DART allows for precise parameterization of simulated images, including exact spatial, spectral, and radiometric resolutions. Also, it enables a 3D parameterization of an agricultural field's optical properties, such as leaves and soil. By training a machine learning algorithm on DART-simulated multispectral images labelled with known input plant biochemical traits, we aim to quantitatively map some of the key biochemical leaf traits, e.g., contents of foliar pigments, with a higher accuracy and greater robustness against confounding factors. We will also explore the potential transferability of this hybrid, i.e. combined physical and non-parametric statistical, approach to other locations with different environmental conditions. Although it has not been tested on real UAS imagery yet, the in-silico results indicate the potential application of the method for estimating chlorophyll contents of individual leaves for maize (Zea mays) crop canopies. Our current efforts include adapting and expanding the method to various maize growth stages, as well as to other economicallyimportant crops, such as sugar beet (Beta vulgaris).
Keywords: 3D radiative transfer, machine learning, high-throughput phenotyping, UAS, multispectral data, leaf biochemical traits
Full title: Combining in-silico multispectral imagery from 3D radiative transfer with machine learning for retrieval of plant biochemical traits.
Abstract: This study presents development of a novel drone-based approach for high-throughput plant phenotyping. This approach uses labelled multispectral imagery generated by the three-dimensional (3D) discrete anisotropic radiative transfer (DART) model, to train a machine learning algorithm retrieving biochemical traits of individual plant leaves from complementary high spatial resolution optical imagery acquired with an uncrewed aircraft system (UAS). Traditional field phenotyping methods are often limited by the need for extensive and invasive sampling practices, costly equipment, and specific technical requirements to capture the complexity of plant responses under controlled or varying environmental conditions. A number of remote sensing phenotyping methods rely on empirical relationships between biochemical traits and vegetation optical indices, calibrated and validated with field measurements. Although some studies have used radiative transfer modelling for phenotyping purposes, they frequently do not account for full 3D structural heterogeneity of complex canopies. Our method addresses the spatial variability in crop traits and the often non-representative nature of field measurements. It is expected to enhance the accuracy and efficiency of phenotyping practices by using the full 3D DART to simulate multispectral data with 1 cm spatial sampling distance, mimicking the UAS images of an off-the-shelf 10-band multispectral sensor from the Micasense company. DART allows for precise parameterization of simulated images, including exact spatial, spectral, and radiometric resolutions. Also, it enables a 3D parameterization of an agricultural field's optical properties, such as leaves and soil. By training a machine learning algorithm on DART-simulated multispectral images labelled with known input plant biochemical traits, we aim to quantitatively map some of the key biochemical leaf traits, e.g., contents of foliar pigments, with a higher accuracy and greater robustness against confounding factors. We will also explore the potential transferability of this hybrid, i.e. combined physical and non-parametric statistical, approach to other locations with different environmental conditions. Although it has not been tested on real UAS imagery yet, the in-silico results indicate the potential application of the method for estimating chlorophyll contents of individual leaves for maize (Zea mays) crop canopies. Our current efforts include adapting and expanding the method to various maize growth stages, as well as to other economicallyimportant crops, such as sugar beet (Beta vulgaris).
Keywords: 3D radiative transfer, machine learning, high-throughput phenotyping, UAS, multispectral data, leaf biochemical traits
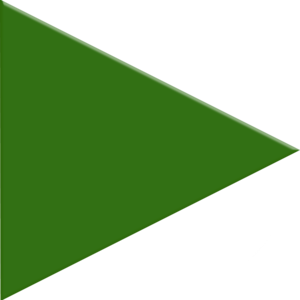
Presented by: Hafiz Ali Imran
Full title: High resolution UAV hyperspectral imaging for monitoring vegetation
Abstract: High-resolution hyperspectral aerial imaging acquired offers a unique view of the vegetation and allows monitoring the health status, identifying specific materials, assessing chemical composition, detecting changes and monitoring phenomena that are not visible in products obtained from standard multispectral imagery. AVT Airborne Sensing Italia (AVT-ASI) uses the hyperspectral sensors AFX10 and AisaFENIX 384 by Specim to acquire hyperspectral images from UAV and airborne platforms for vegetation analysis and has developed a workflow for identifying plant diseases, mapping invasive species, bark beetle damage, calculating narrowband vegetation indices, and analyzing overall health status. The poster will highlight the challenges of acquiring UAV hyperspectral images, including factors such as flight duration, flight direction, and environmental conditions. Some preliminary results will be discussed on the detection of Flavescenza dorata in vineyards and apple proliferation in apple orchards in Trento area using UAV hyperspectral images, demonstrating the ability to early detection of infected plants. Finally, findings from a forest health mapping project in South Tyrol will be presented, where narrowband indices were used to assess forest condition and detect areas impacted by stress or disease. These projects highlight the potential of hyperspectral aerial imaging as a powerful tool for advancing precision agriculture and forest management.
Keywords: UAV Hyperspectral imaging, plant diseases, forest health
Full title: High resolution UAV hyperspectral imaging for monitoring vegetation
Abstract: High-resolution hyperspectral aerial imaging acquired offers a unique view of the vegetation and allows monitoring the health status, identifying specific materials, assessing chemical composition, detecting changes and monitoring phenomena that are not visible in products obtained from standard multispectral imagery. AVT Airborne Sensing Italia (AVT-ASI) uses the hyperspectral sensors AFX10 and AisaFENIX 384 by Specim to acquire hyperspectral images from UAV and airborne platforms for vegetation analysis and has developed a workflow for identifying plant diseases, mapping invasive species, bark beetle damage, calculating narrowband vegetation indices, and analyzing overall health status. The poster will highlight the challenges of acquiring UAV hyperspectral images, including factors such as flight duration, flight direction, and environmental conditions. Some preliminary results will be discussed on the detection of Flavescenza dorata in vineyards and apple proliferation in apple orchards in Trento area using UAV hyperspectral images, demonstrating the ability to early detection of infected plants. Finally, findings from a forest health mapping project in South Tyrol will be presented, where narrowband indices were used to assess forest condition and detect areas impacted by stress or disease. These projects highlight the potential of hyperspectral aerial imaging as a powerful tool for advancing precision agriculture and forest management.
Keywords: UAV Hyperspectral imaging, plant diseases, forest health
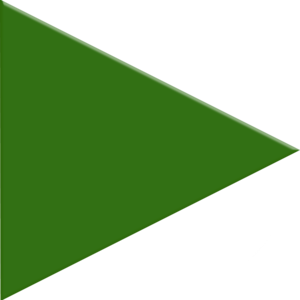
Presented by: Vicente Burchard Levine
Full title: Examining the relationship between spectral diversity, functional diversity and water use efficiency in a semi-arid grassland using field spectroscopy and high-resolution UAV imagery
Abstract: Vegetation diversity has been demonstrated to influence ecosystem function, providing essential ecosystem services. However, the biodiversity-ecosystem function (BEF) relationship is very complex and still not fully accounted for at different spatial-temporal scales. Remote sensing has gained traction as one of the one only viable methods to monitor plant diversity at different scales, most commonly exploiting the spectral variability hypothesis (SVH), which relates spectral heterogeneity to plant diversity. This study focused on examining the relationship between functional diversity (FD), spectral diversity (SD) and water use efficiency (WUE) of the herbaceous understory of a Mediterranean tree-grass ecosystem (TGE) using field spectroscopy and high resolution imagery from unmanned aerial vehicles (UAVs). Multiple field campaigns were performed in 2021 and 2023 at the Majadas de Tiétar experimental eddy covariance (EC) station located in Spain to collect in-situ measurements of functional traits, hyperspectral field spectroscopy and high-resolution visible-near-infrared (VNIR) and thermal infrared (TIR) imagery onboard UAVs. Significant relationships were found between spectral diversity and functional diversity across different phenological periods using a canopy-scale spectral library while the UAV overpasses revealed a significant positive relationship between SD metrics based on VNIR indices and FD metrics calculated using more traditional ecologically-based methods. Along with this, preliminary results combining VNIR and TIR imagery to estimate WUE using UAVs through an energy balance approach will be presented and compared to the SD and FD metrics.
Poster Dataset: Poster PDF
Return to workshop programFull title: Examining the relationship between spectral diversity, functional diversity and water use efficiency in a semi-arid grassland using field spectroscopy and high-resolution UAV imagery
Abstract: Vegetation diversity has been demonstrated to influence ecosystem function, providing essential ecosystem services. However, the biodiversity-ecosystem function (BEF) relationship is very complex and still not fully accounted for at different spatial-temporal scales. Remote sensing has gained traction as one of the one only viable methods to monitor plant diversity at different scales, most commonly exploiting the spectral variability hypothesis (SVH), which relates spectral heterogeneity to plant diversity. This study focused on examining the relationship between functional diversity (FD), spectral diversity (SD) and water use efficiency (WUE) of the herbaceous understory of a Mediterranean tree-grass ecosystem (TGE) using field spectroscopy and high resolution imagery from unmanned aerial vehicles (UAVs). Multiple field campaigns were performed in 2021 and 2023 at the Majadas de Tiétar experimental eddy covariance (EC) station located in Spain to collect in-situ measurements of functional traits, hyperspectral field spectroscopy and high-resolution visible-near-infrared (VNIR) and thermal infrared (TIR) imagery onboard UAVs. Significant relationships were found between spectral diversity and functional diversity across different phenological periods using a canopy-scale spectral library while the UAV overpasses revealed a significant positive relationship between SD metrics based on VNIR indices and FD metrics calculated using more traditional ecologically-based methods. Along with this, preliminary results combining VNIR and TIR imagery to estimate WUE using UAVs through an energy balance approach will be presented and compared to the SD and FD metrics.
Poster Dataset: Poster PDF